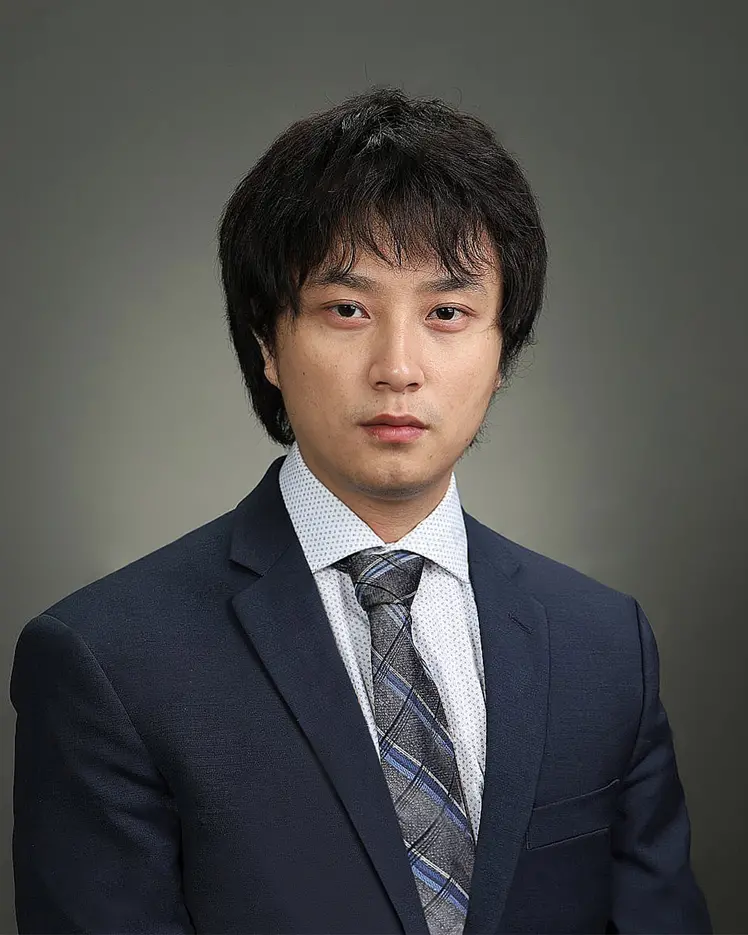
Bang Liu
Biography
Bang Liu is an assistant professor in the Department of Computer Science and Operations Research (DIRO), and a core member of the Applied Research in Computational Linguistics Lab (RALI) at Université de Montréal. He is also an associate academic member of Mila – Quebec Artificial Intelligence Institute and a Canada CIFAR AI Chair.
Liu received his BEng from the University of Science and Technology of China in 2013, and his MSc and PhD degrees from the University of Alberta in 2015 and 2020, respectively. His research interests lie primarily in the areas of natural language processing, multimodal and embodied learning, theory and techniques for AGI (e.g., understanding and improving large language models), and AI for science (e.g., health, material science, XR).