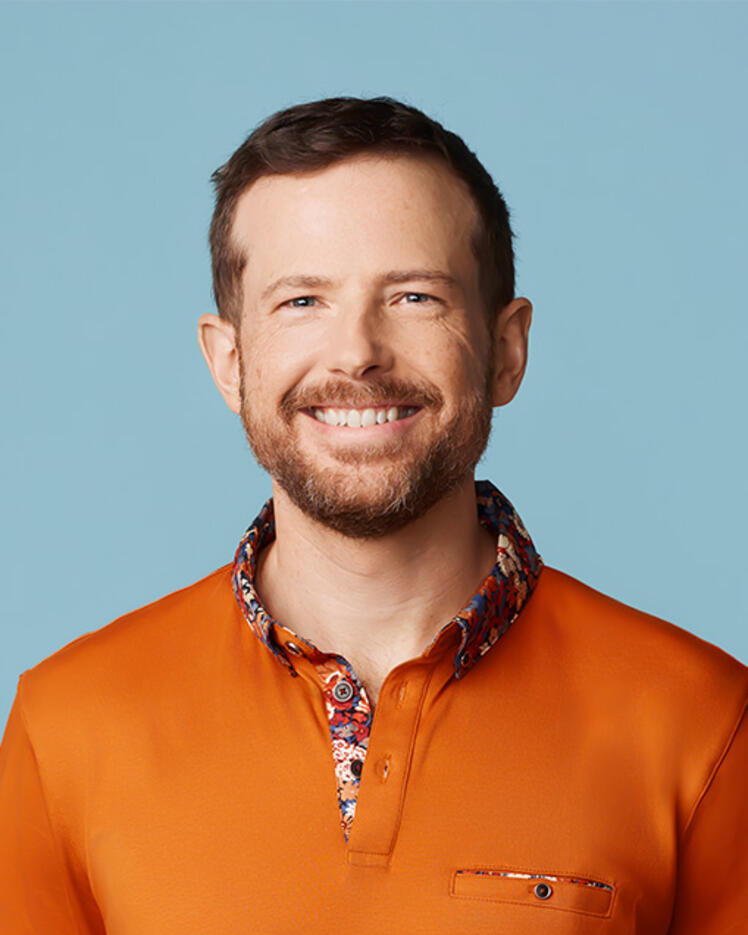
Blake Richards
Biography
Blake Richards is an associate professor at the School of Computer Science and in the Department of Neurology and Neurosurgery at McGill University, and a core academic member of Mila – Quebec Artificial Intelligence Institute.
Richards’ research lies at the intersection of neuroscience and AI. His laboratory investigates universal principles of intelligence that apply to both natural and artificial agents.
He has received several awards for his work, including the NSERC Arthur B. McDonald Fellowship in 2022, the Canadian Association for Neuroscience Young Investigator Award in 2019, and a Canada CIFAR AI Chair in 2018. Richards was a Banting Postdoctoral Fellow at SickKids Hospital from 2011 to 2013.
He obtained his PhD in neuroscience from the University of Oxford in 2010, and his BSc in cognitive science and AI from the University of Toronto in 2004.