Home
Inspiring the development of artificial intelligence for the benefit of all
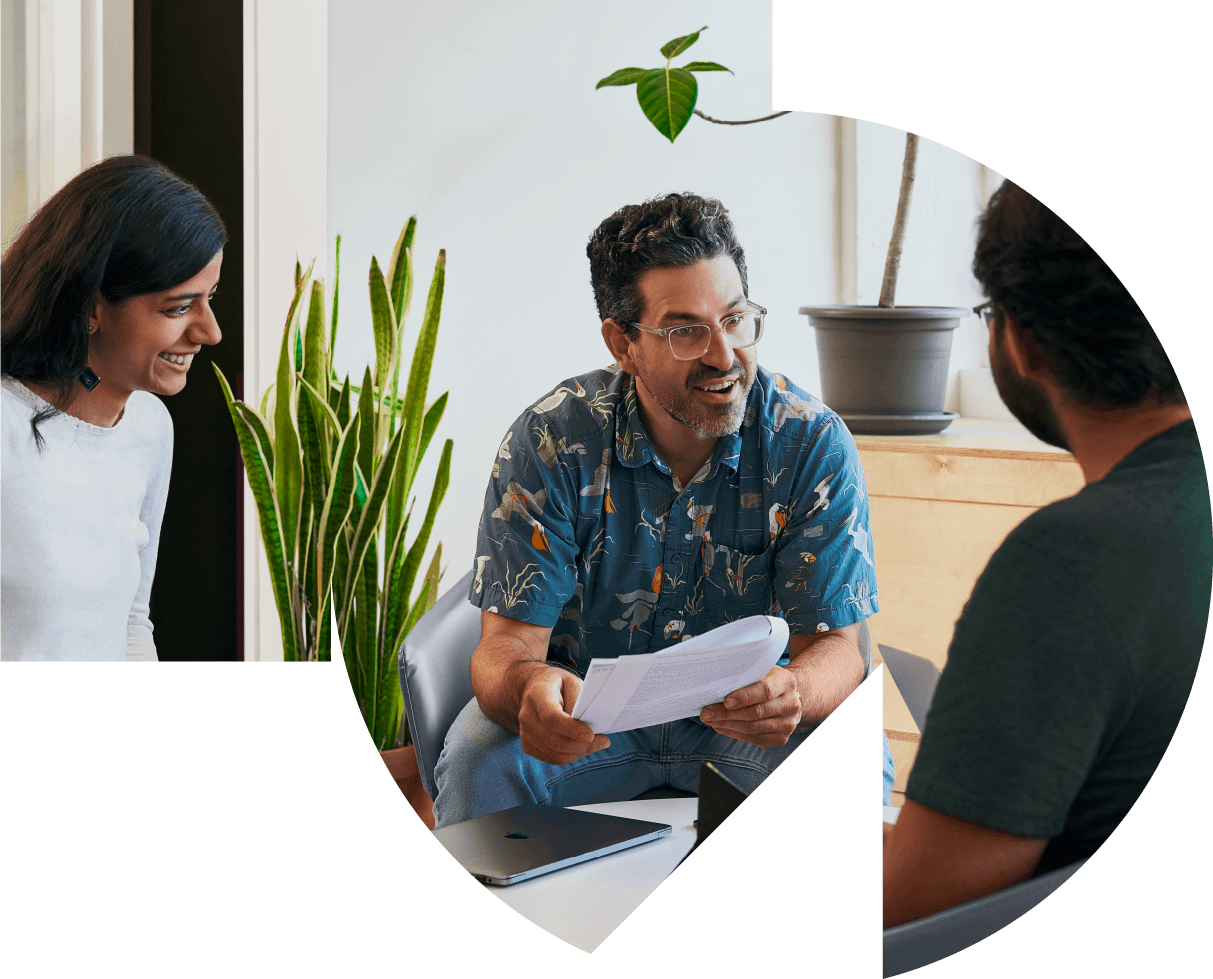
Founded in 1993 by Professor Yoshua Bengio, Mila today brings together over 140 professors affiliated with Université de Montréal, McGill University, Polytechnique Montréal and HEC Montréal. Mila also welcomes professors from Université Laval, Université de Sherbrooke, École de technologie supérieure (ÉTS) and Concordia University.
Socially responsible and beneficial development of AI is a fundamental component of Mila’s mission. As a leader in the field, we wish to contribute to social dialogue and the development of applications that will benefit society.