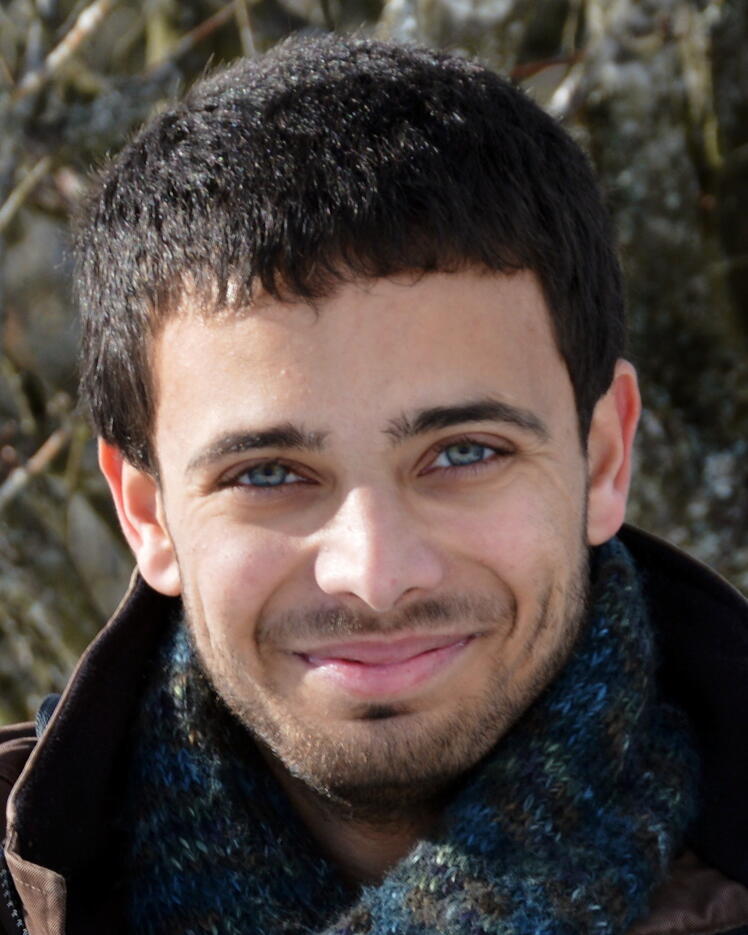
Quentin Cappart
Biography
Quentin Cappart is an associate professor in the Department of Computer and Software Engineering at Polytechnique Montréal and an Affiliate member at Mila. He leads the CORAIL research group, which he co-founded with Louis-Martin Rousseau. Cappart obtained a BSc in engineering (2012), a MSc in computer engineering (2014), a MSc in management (2018) and a PhD (2017) at the Université catholique de Louvain (Belgium).
After his PhD, he joined Polytechnique Montréal and the International Research Centre on Enterprise Networks, Logistics and Transportation (CIRRELT) as a postdoctoral fellow (2018–2020). During these two years, he was also a research intern at ElementAI. Cappart’s main research area is the integration of machine learning with search procedures for solving combinatorial problems.