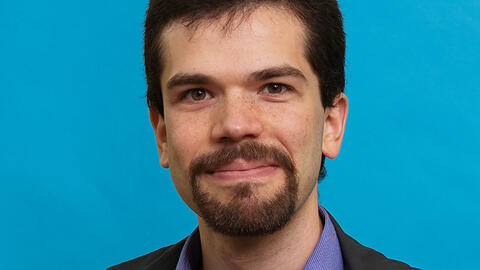
In February 2022, Mila researchers and experts from around the world published the much anticipated report “Tackling Climate Change with Machine Learning” in ACM Computing Surveys. In it, they discuss how machine learning (ML) can be a powerful tool to help reduce greenhouse gas emissions and help society adapt to climate change, one of the greatest threats facing humanity.
We had the opportunity to discuss the report with the lead author, Mila Professor David Rolnick—a pioneer at the intersection of ML and climate change—Canada CIFAR AI Chair, Assistant Professor in the School of Computer Science at McGill University and a Co-Founder and Chair of Climate Change AI. Below, Rolnick provides an overview of the report, some of the problem areas that can be addressed by ML, and the promising research and business opportunities ahead as the world gathers to tackle climate change.
Q. What are the report’s central themes, and who are you specifically addressing?
A. The report is aimed at researchers and practitioners, both in machine learning and in areas such as energy, agriculture, urban planning, and climate policy. We provide a detailed taxonomy of areas of climate action where ML can be valuable, as well as a roadmap for working at the intersection of ML and climate change. The report emphasizes that impactful work requires close collaboration between different stakeholders. This is essential to ensure that the right problems are being solved; that ML algorithms incorporate relevant domain knowledge; and that there is a pathway to deployment. We also stress that ML is not a silver bullet in climate action—it is merely one tool in the fight against climate change, and it is impactful only when used in combination with tools from other fields or sectors.
Q. In the report, the authors outline machine learning applications that could have immediate impacts in the fight against climate change and others that could be undertaken further down the road. What are some of the applications that could be implemented in the short-term?
A. We highlight a number of overarching themes for how ML can be impactful in climate action. ML can turn raw data into useful information—for example, by using satellite imagery to automatically pinpoint deforestation or identify risks from coastal flooding or by parsing corporate financial reports to identify climate-related disclosures. ML can optimize complex systems - for example, by reducing the energy needed to heat and cool buildings or by boosting the efficiency of freight transportation networks. ML can improve forecasting, for instance, by predicting supply and demand to help manage electrical grids, or by forecasting crop yields. And ML can accelerate the process of scientific modeling via, for instance, fast approximation of certain pieces of climate and weather models that are otherwise very time-intensive to compute.
Q. Could you provide an example of one ML application that may take longer to implement, but that may have a significant impact?
A. We are eager to see applications of ML to help accelerate the scientific discovery of new materials like those needed for batteries, perovskite solar cells, catalysts for energy-intensive industrial processes, and more. In these cases, ML can learn from the results of past experiments to suggest candidate materials for experimentation. It’s not a replacement for manual lab work, but it has the potential to reduce the number of experiments that need to be tried and therefore speed up the process of experimentation. My group and others at Mila are already working on ML for catalyst discovery, and outside of the climate space there is related work focused on drug discovery.
Q. What are some of the potentially exciting business opportunities ahead within the scope of ML and climate change?
A. Many, if not most, of the applications we highlight in the paper have the potential to be developed and deployed scalably in the private sector. Some especially exciting opportunities lie in heavy industries such as cement and steel manufacturing, where there is the potential for significant improvements in energy efficiency and greenhouse gas emissions reduction. Often these industries are slow to adopt new technologies (including because they need strong reliability guarantees), but dedicated entrepreneurs and ML practitioners who work closely with these players and respond to their needs can potentially have a big impact.
Q. Are there risks involved in ML applications in relation to climate change?
A. Overall, ML can pose significant risks as well as opportunities in relation to climate change. One risk is technosolutionism—that shiny new technological tools like ML can divert attention or funding from other strategies for climate action. Additional risks are associated with equity; for example, ML tools and data are often disproportionately controlled by certain institutions and regions and can thereby serve to exacerbate power imbalances and digital divides. ML can also make climate change worse, e.g., the widespread applications of ML to accelerate fossil fuel extraction, the emissions associated with hardware and computation, and the broader effects of ML-based advertising on societal consumption patterns.
Q. Could you tell us about one of the strategies outlined in the report that you are currently focusing on?
A. One of the areas of work that we are currently focusing on is physics-informed ML. There are many climate-relevant problems where we know the solution has to have certain properties, and we want to make sure that ML algorithms respect those properties. For example, we’ve been working on using deep learning to approximately solve certain hard optimization problems in electrical grid management. We’ve worked out a way to build physics-based constraints associated with the electrical grid into the deep learning system, which prevents the system from proposing impractical “solutions” that would lead to a blackout. We’re also working on using this kind of approach in other contexts, like deep learning for modeling certain kinds of atmospheric physics, where again there are known rules that shouldn’t be violated (like conservation of mass or energy).
To read the report and for the full list of authors, click here.
Tackling Climate Change with Machine Learning: Mila Contributors
- Yoshua Bengio, Founder & Scientific Director, Mila, Full Professor, Université de Montréal
- David Rolnick, Assistant Professor, McGill University, Mila
- Sasha Luccioni, Mila postdoc, Research Scientist, HuggingFace
- Tegan Maharaj, Mila Alum, Assistant Professor, Faculty of Information, University of Toronto
- Kris Sankaran, Mila Alum, Assistant Professor of Statistics, University of Wisconsin-Madison