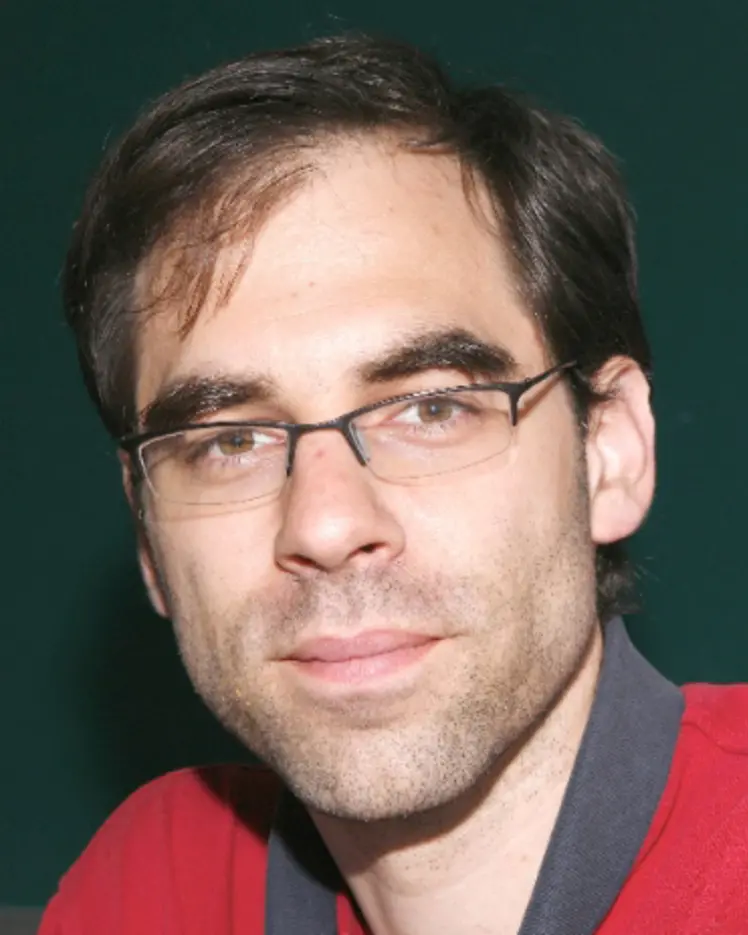
Mathieu Blanchette
Biography
Mathieu Blanchette is an associate professor and director of the School of Computer Science at McGill University.
After completing his PhD (University of Washington, 2002) and a postdoc (UC Santa Cruz, 2003), Blanchette joined the School of Computer Science at McGill, where he founded the Computational Genomics Lab. Research carried out in this lab has resulted in more than seventy publications.
Blanchette is an elected member of the College of New Scholars, Artists and Scientists of the Royal Society of Canada and a 2009 Sloan Research Fellow. He was awarded the Outstanding Young Computer Scientist Researcher Prize by the Canadian Association for Computer Science in 2012, and the Overton Prize in 2006.
He loves teaching and supervising students, for which he was honoured with McGill’s Leo Yaffe Award for Excellence in Teaching (2008).