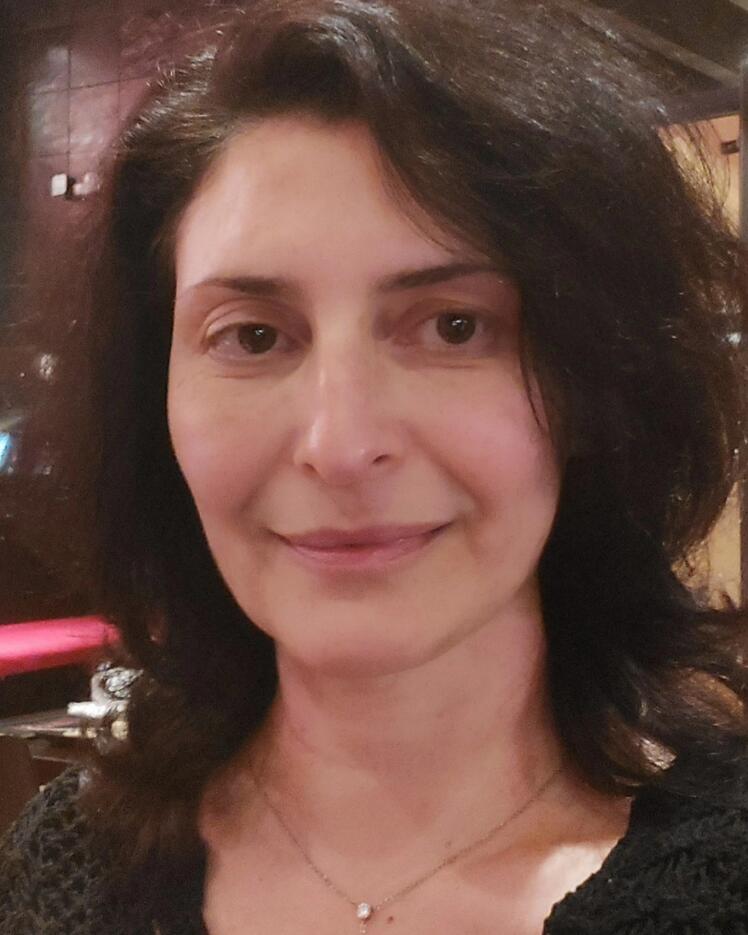
Irina Rish
Biography
Irina Rish is a full professor at the Université de Montréal (UdeM), where she leads the Autonomous AI Lab, and a core academic member of Mila – Quebec Artificial Intelligence Institute.
In addition to holding a Canada Excellence Research Chair (CERC) and a CIFAR Chair, she leads the U.S. Department of Energy’s INCITE project on Scalable Foundation Models on Summit & Frontier supercomputers at the Oak Ridge Leadership Computing Facility. She co-founded and serves as CSO of Nolano.ai.
Rish’s current research interests include neural scaling laws and emergent behaviors (capabilities and alignment) in foundation models, as well as continual learning, out-of-distribution generalization and robustness.
Before joining UdeM in 2019, she was a research scientist at the IBM T.J. Watson Research Center, where she worked on various projects at the intersection of neuroscience and AI, and led the Neuro-AI challenge. She was awarded the IBM Eminence & Excellence Award and IBM Outstanding Innovation Award (2018), IBM Outstanding Technical Achievement Award (2017) and IBM Research Accomplishment Award (2009).
She holds 64 patents and has published 120 research papers, several book chapters, three edited books and a monograph on sparse modeling.