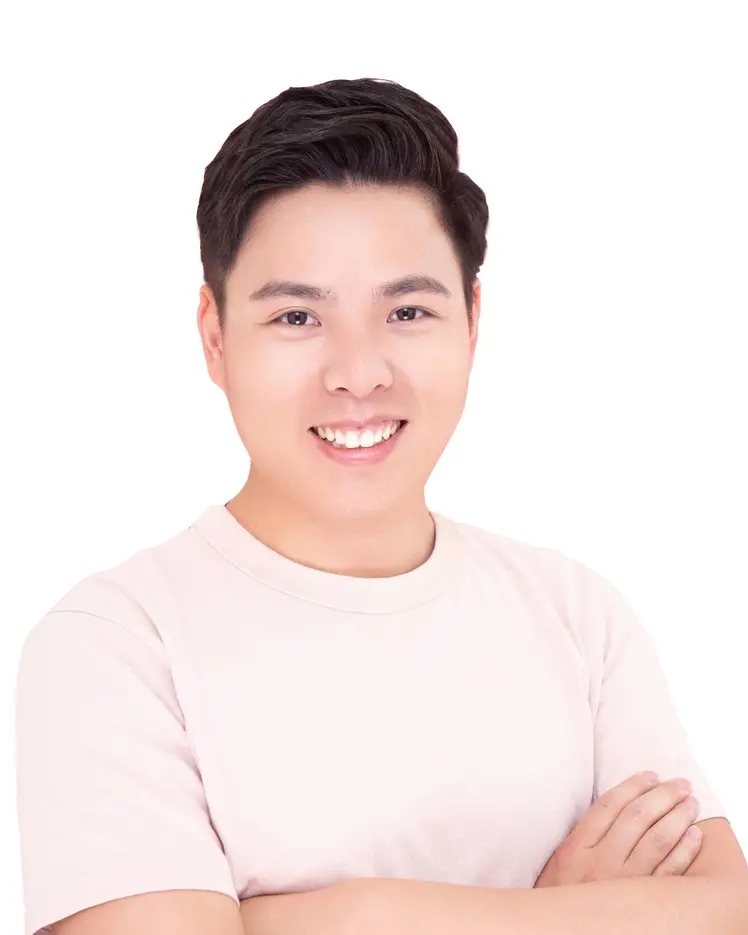
Jian Tang
Biography
Jian Tang is an Associate professor at HEC's Department of Decision Sciences. He is also an Adjunct professor at the Department of Computer Science and Operations Research at University of Montreal and a Core Academic member at Mila - Quebec AI Institute. He is a Canada CIFAR AI Chair and the Founder of BioGeometry, an AI startup that focuses on generative AI for antibody discovery. Tang’s main research interests are deep generative models and graph machine learning, and their applications to drug discovery. He is an international leader in graph machine learning, and LINE, his node representation method, has been widely recognized and cited more than five thousand times. He has also done pioneering work on AI for drug discovery, such as developing the first open-source machine learning frameworks for drug discovery, TorchDrug and TorchProtein.