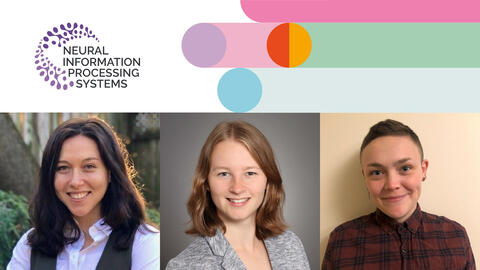
AI can be a powerful tool to detect discriminatory behavior online, but for years, tackling gender bias and misogynistic undertones has been a challenge for machine learning researchers. To bridge that gap, a team at Mila has been working since 2021 on a novel open-source dataset to help detect, quantify and reduce subtle misogynistic text by working with an interdisciplinary team of machine learning researchers, social sciences experts and annotators.
Subtle Misogyny Detection and Mitigation: An Expert-Annotated Dataset, accepted as a spotlight submission and oral presentation at a NeurIPS 2023 workshop, proposes a dataset meant to facilitate the automated detection and removal of subtle misogyny in text. This recognition is a milestone for the Biasly project that started more than four years ago.
Two of the co-authors, Anna Richter and Brooklyn Sheppard, natural language processing (NLP) scientists at Mila with backgrounds in cognitive science and linguistics, joined the project in 2022 with an aim to create a dataset to spot and remove subtle instances of misogyny in text.
“When people think about misogyny, they often have very strong forms in mind, but there is also another form of misogyny which is much more subtle, but also much more present in everyday life of many of us women in our society,” Anna Richter said.
Allison Cohen, co-author on the paper and leading the project at Mila, explained that about twenty people have joined the project since 2021, from machine learning researchers to annotators to experts in social sciences.
She said that the project is an opportunity to establish a dialogue between various disciplines and to value the perspectives of annotators to ensure that the dataset is effective, trustworthy, and developed responsibly.
“So much of the quality of the work depends on the process,” she emphasized.
Interdisciplinary teamwork
Datasets to detect stronger forms of misogyny do exist, including hate speech and slur detection more broadly, but the researchers identified a lack of high-quality datasets to tackle more subtle instances.
Doing so, however, required deeper expertise in other fields, including social sciences.
They thus collaborated with a team of interdisciplinary experts in gender studies (Tamara Kneese) and linguistics (Elizabeth Smith) as well as an NLP advisor (Yue Dong) to ensure that the dataset was as robust as possible. All the researchers, experts and most annotators were women, and all annotators also had a background in these domains.
"It's not your typical team for a technical paper, that's for sure," Brooklyn Sheppard quipped.
Insights from interdisciplinary experts were crucial in the creation of the database, she said.
"Our gender studies expert helped us identify the types of misogyny, and the linguistics expert came into play when the time came to re-writing the misogynistic text to make it non-misogynistic while keeping the overall meaning intact," she explained.
The dataset was built using movie subtitle data from North American films from the past 10 years.
"Over an iterative process and dialogue between the machine learning and social science sides of the team, we were able to find an optimal way of sampling the keywords such that we get enough misogyny, but don't bias the data set in a too strong way," Anna Richter explained.
Raising awareness in the machine learning community
She said that being given a spotlight recognition at NeurIPS proves that the machine learning community is interested in doing AI research more responsibly.
"Being given this spotlight recognition and the oral recognition really is a sign to us that the machine learning community wants more of this slower, detail-oriented, interdisciplinary, ethical approach to doing things," Anna Richter said.
"I really hope that this paper will make the machine learning community aware of the work that is needed to create a high quality dataset. We have detailed the process so that other people who also want to create a dataset, in particular for more socio-technical problems with a lot of subjectivity involved, can do so," she added.
She emphasized the ethical dimension of the process: the annotators were compensated fairly, provided with mental health support and benefited from regular check-ins with the team.
Brooklyn Sheppard said that modern AI development can only benefit from interdisciplinary collaborations.
"It is very important at this stage in AI in general to have social scientists on board, to have people from other disciplines analyzing these models, helping to create these models because they are getting so powerful that without those checks and that ethical focus, it can get very dangerous,” she emphasized.
"I would also like to encourage other women who are thinking about going into AI to do so. There are projects like ours where you have an awesome team of extremely capable, extremely motivated women working on something that is beneficial for society," she added.
Biasly started out in 2019 as a prototype led by Andrea Jang, Carolyne Pelletier, Ines Moreno and Yasmeen Hitti of the AI4GoodLab, now led by Mila. It has since grown to be a part of the Mila AI for Humanity team’s portfolio of applied projects.