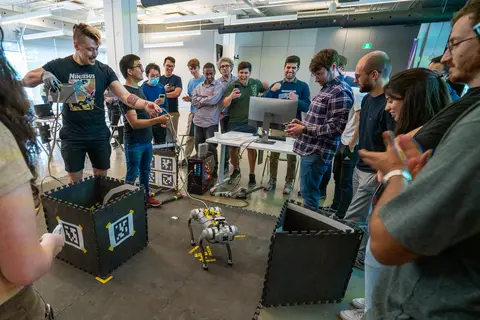
In mid-June 2023, the second edition of Mila’s Robotics Summer School brought together 27 participants who learned about traditional and artificial intelligence robotics methods before applying this knowledge in a competition featuring a four-legged robot navigating through an obstacle course.
During the course of a week, participants got familiar with state-of-the-art robotics methods, learned about the latest research on machine learning techniques and worked on hands-on projects, also known as “robot time.”
Glen Berseth, Core Academic Member at Mila and Assistant Professor at Université de Montréal, explained that the Summer School seeks to bridge the gap between traditional robotics methods and machine learning models that can improve the adaptability of robots in challenging situations.
The School was created last year to bring together a group of machine learning specialists wanting to apply their skills to real hardware and match them with a group of specialists in robotics wanting to learn more about AI applications in their field.
“Machine learning works better than normal robotics models whenever things like physics and dynamics are not linear and very hard to model. Often this happens in areas where the robot is interacting with its environment, this is where it is fun and interesting,” Glen Berseth explained.
This is especially true in the case of the four-legged robot used during the Summer school, which has more complex and unpredictable dynamics than simpler and slower hardware with wheels, for instance.
“Legged robots can do a lot of exciting jumping and running,” he said.
With enough data, machine learning methods can thus be used to approximate these situations and prepare the robot to deal with an unstable environment.
Training for unpredictability
To prepare for the competition at the end of the program, participants used deep reinforcement learning to pre-train policies and machine learning models in simulations, Glen Berseth explained.
« partcipants have a simulated version of the actual hardware so that they can -in a much more speedy manner- train a policy that can control the simulated version and then transfer that onto the real hardware. They can then compare how well the model works on the real hardware versus traditional robotics methods. »
Florian Golemo, a postdoctoral student at Mila who was in charge of operationalizing robotics exercises and coaching participants throughout the program, said that students used a state-of- the-art simulator called Isaac Gym to train complicated robot tasks in minutes.
“The robot comes with a classic controller, but it has large issues when going over obstacles or doing anything slightly unexpected. If the robot is in this mode and you try to lift it up, it will freak out and its legs will go in all directions,” Golemo explained.
But “once you train the robot with deep reinforcement learning, it is much more robust. In our simulator, we have a bunch of different terrains like steps, bumpy or bouncy terrain, and the robot is ready to walk over all of these in half an hour, where it would take weeks or months to design the classic controller.”
Most of all, he liked how passionate students were, spending late nights crafting their robot projects ahead of the competition.
“The students got really invested in the competition, cheering on their robot like it was a football team!,” he recalled.
Novel ideas
Sacha Morin, a PhD student at Mila, taught participants how to coordinate information from multiple sensors across the robot, especially cameras, to plan a trajectory and avoid obstacles. Over the course of the week, he witnessed the “impressive” progress of students.
“On the first two days, the participants were learning their environment, understanding the code, and the robot wasn't doing much. But over the following days, movement and navigation really improved. You could see that the summer school was paying off, but also the magic of deadlines as the competition approached, with everyone putting their heart and soul in it.”
“What I really like about robotics is seeing your program and ideas come to life. I was happy to be able to share this experience with other students, especially those with more of a machine learning background and less familiar with robotics.”
Glen Berseth concluded by emphasizing novel ideas brought by new to machine learning for robotics to solve problems.
« I liked the very unique ideas we ended up getting from some of the students… They came up with some really interesting ideas about how to be able to program or use machine learning methods to control the hardware and accomplish the competition tasks at the end of the week. »
He now hopes to see the Summer School grow in the next few years, with more hardware, more students and more hands-on time, as well as establishing partnerships with local companies to showcase the very latest robotics hardware to budding researchers.