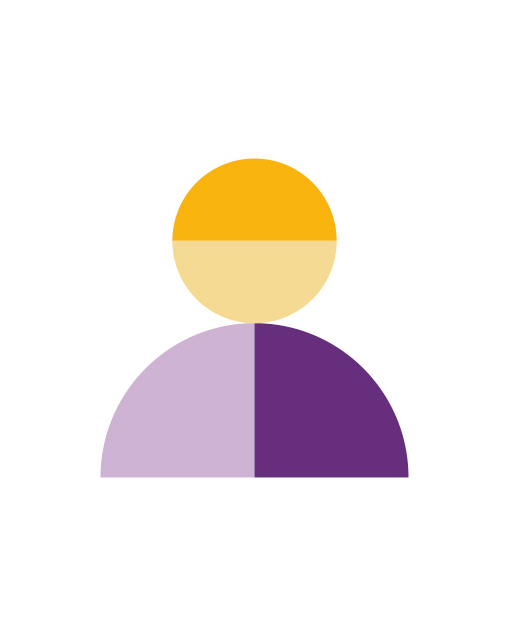
Gregory Dudek
Biography
Gregory Dudek is a full professor at McGill University’s CIM which is linked to the School of Computer Science and Research Director of Mobile Robotics Lab. He is also the Lab Director and VP of research at Samsung AI Center Montreal and an Associate academic member at Mila - Quebec Institute of Artificial Intelligence.
Dudek has authored and co-authored over 300 research publications on a wide range of subjects, including visual object description, recognition, RF localization, robotic navigation and mapping, distributed system design, 5G telecommunications and biological perception.
He co-authored the book “Computational Principles of Mobile Robotics” (Cambridge University Press) with Michael Jenkin. He has chaired and been involved in numerous national and international conferences and professional activities concerned with robotics, machine sensing and computer vision.
Dudek’s research interests include perception for mobile robotics, navigation and position estimation, environment and shape modelling, computational vision and collaborative filtering.