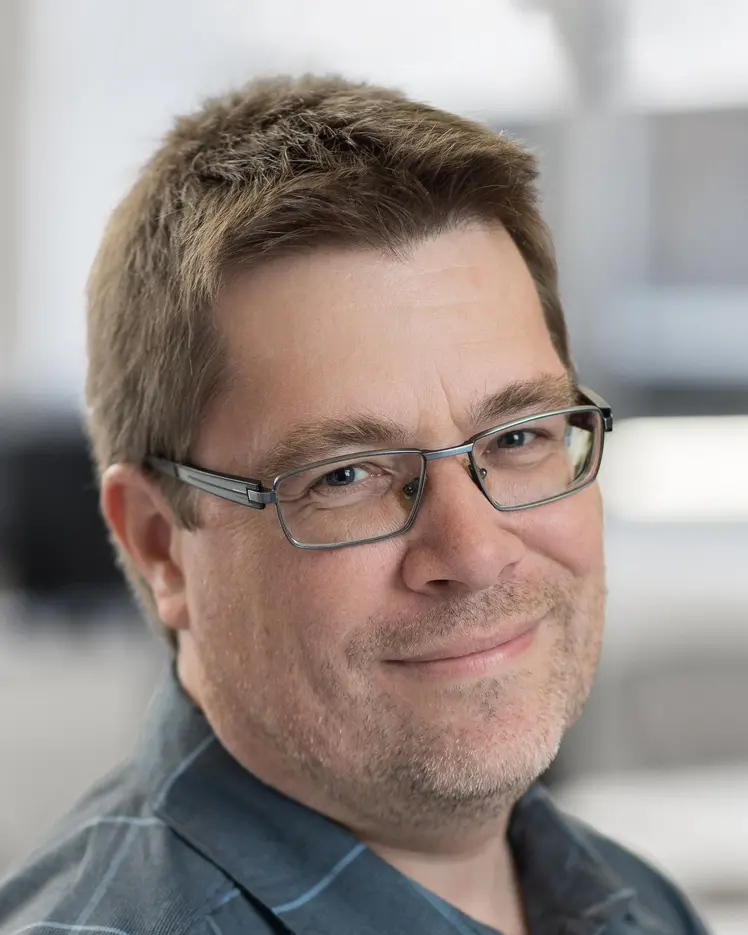
Pascal Vincent
Biography
Pascal Vincent is a research scientist in the Fundamental AI Research (FAIR) team at Meta and an adjunct professor in the Department of Computer Science and Operations Research (DIRO) at Université de Montréal.
He is also a founding member of Mila – Quebec Artificial Intelligence Institute and an associate fellow in CIFAR’s Learning in Machines & Brains program.
Vincent’s research on principles and algorithms in representation learning led him to uncover several seminal ideas that became key enablers for the successes of deep learning methods. Among his most influential contributions is the seminal paper on neural language models “A Neural Probabilistic Language Model” (Bengio et al. 2013), which laid the foundations on which all artificial neural network based language models are built.
His work on denoising autoencoders (Vincent et al. 2008, 2010) was the first to propose the pretext task of filling in artificially introduced blanks for the sake of learning useful representations in any modality, a precursor of what is today called self-supervised learning.
In another seminal paper, “A Connection Between Score Matching and Denoising Autoencoders” (Vincent 2011), he developed the “denoising score matching” principle, which is now routinely used to train diffusion-based generative models.
Vincent’s current research focuses on novel theory and algorithms for representation learning to enable robust generalization out-of-distribution.