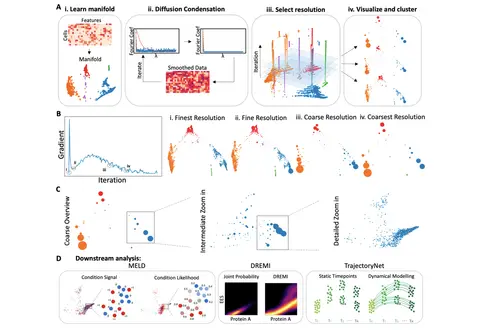
Last spring, Guy Wolf, Mila core academic member and Assistant Professor at Université de Montréal teamed up with Smita Krishnaswamy (Yale), Julie Hussin (MHI & UdeM), Martin Smith (CHUSJ & UdeM), Morgan Craig (CHUSJ & UdeM) and several other leading experts to launch the genomics taskforce to help find potential solutions to the ongoing pandemic. Their latest findings indicate interesting patterns that may form disease signatures and predict patient outcome.
While the accelerating data deluge in biology and medicine is evident, so is the urgency to develop methods for visualizing and analyzing increasingly massive data at multiple resolutions. As such, the focus of this particular COVID-19 taskforce is on medical data collection combined with statistical machine learning analysis in order to achieve a better understanding of COVID-19 disease progression.
“One of the main goals we have is to support efforts to understand the progression and development of the COVID-19 disease, improve diagnosis procedures and treatment regiments, which all require, or at least could benefit from, effective and efficient processing of big data, especially in the bioinformatics domain,” said Professor Wolf.
As a result, the team developed Multiscale PHATE, a new multiresolution visualization method that can learn and visualize abstract cellular features and groupings. Their method was used to process and analyze 54 million cells from 168 patients hospitalized with the infectious disease at the Yale New Haven Hospital in Connecticut. They found that granulocytes and monocytes were most enriched in patients that died from infection, while T cells were most enriched in patients who survived.
The study is undergoing peer-review for publication in Cell and is available as preprint on biorxiv. Short versions of the work were also presented in the Women in Machine Learning and Learning Meaningful Representations of Life workshops, as part of the annual conference on Neural Information Processing Systems (NeurIPS) this past week.
“I think the entire PHATE methodology is very exciting. It is this idea that you can take data in thousands of dimensions and reduce it to 2-3 dimensions in such a way that you preserve the manifold geometry of the data, which can lead to insights about the underlying data generating process,” explained Dr. Smita Krishnaswamy, founder of the Krishnaswamy Lab at Yale University, adding that “each step of PHATE is carefully tuned at preserving manifold geometry and eliminating noise, in a way that other commonly used methods of visualization are not.”
Follow up work is currently being conducted in collaboration with Dr. Julie Hussin to analyze inter- and intra-host genetic variations of SARS-CoV-2. Further interdisciplinary work is planned with researchers from the Centre hospitalier universitaire Sainte-Justine (CHUSJ) and the Centre hospitalier de l’Université de Montréal (CHUM). Finally, the team is expecting to have access to data from clinical trials that they intend to process once they become available
For more information:
The preprint version of the study is available here.
The Multiscale PHATE package is available for download with a guided tutorial on the Krishnaswamy Lab GitHub page: https://github.com/KrishnaswamyLab/Multiscale_PHATE