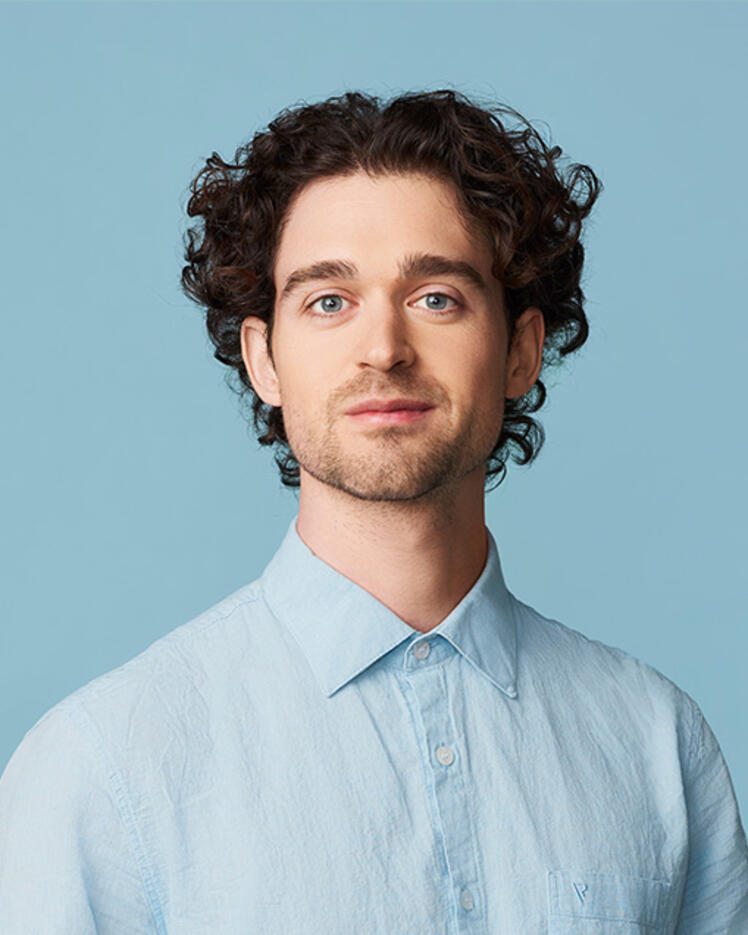
Gauthier Gidel
Biographie
Je suis professeur adjoint au Département d’informatique et de recherche opérationnelle (DIRO) de l'Université de Montréal et membre académique principal de Mila – Institut québécois d’intelligence artificielle. J'ai obtenu une bourse Borealis AI destinée aux étudiant·e·s des cycles supérieurs et je suis actuellement titulaire d'une chaire en IA Canada-CIFAR. J'ai travaillé chez DeepMind et Element AI, et j'ai récemment été un visiteur de longue durée au Simons Institute de l’Université de Californie à Berkeley. Mes intérêts de recherche se situent à l'intersection de la théorie des jeux, de l'optimisation et de l'apprentissage automatique.