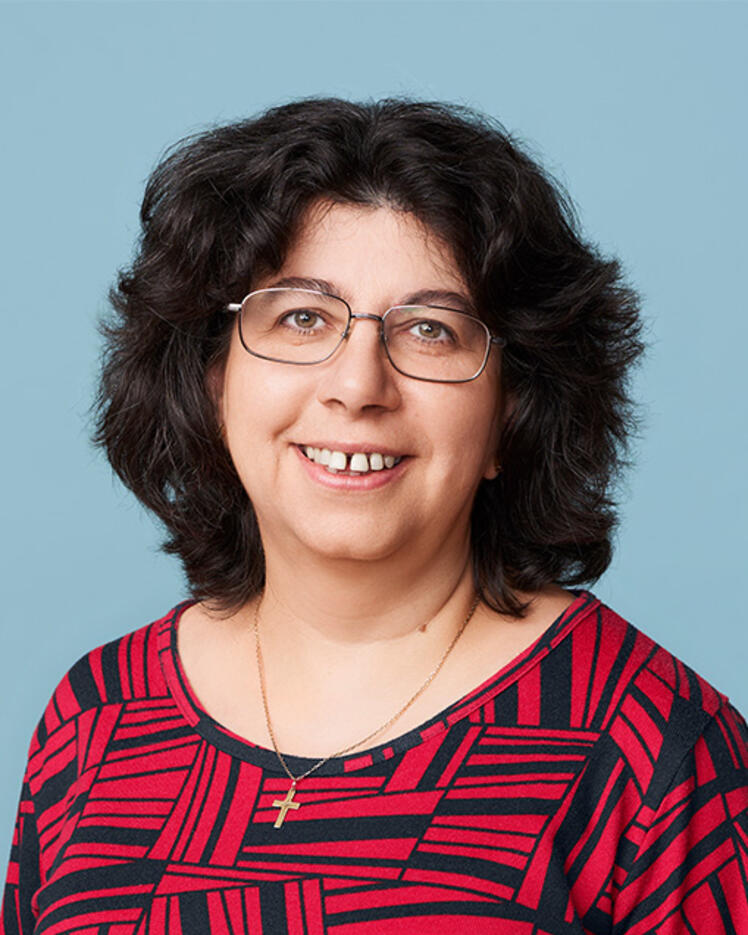
Doina Precup
Biographie
Doina Precup enseigne à l'Université McGill tout en menant des recherches fondamentales sur l'apprentissage par renforcement, notamment les applications de l'IA dans des domaines ayant des répercussions sociales, tels que les soins de santé. Elle s'intéresse à la prise de décision automatique dans des situations d'incertitude élevée.
Elle est membre de l'Institut canadien de recherches avancées (CIFAR) et de l'Association pour l'avancement de l'intelligence artificielle (AAAI), et dirige le bureau montréalais de DeepMind.
Ses spécialités sont les suivantes : intelligence artificielle, apprentissage machine, apprentissage par renforcement, raisonnement et planification sous incertitude, applications.