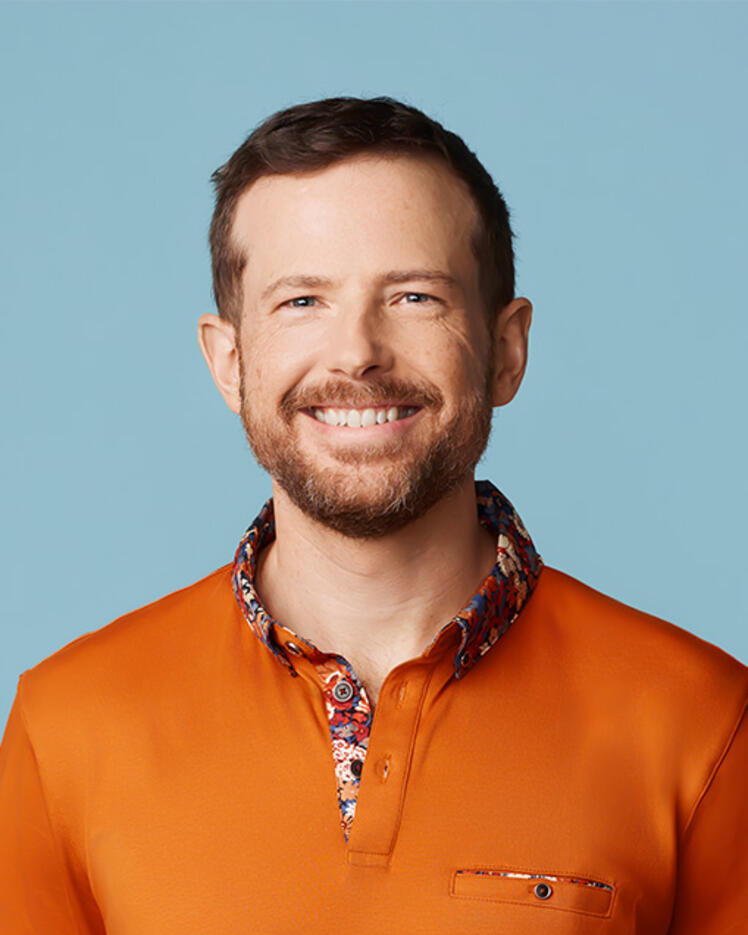
Blake Richards
Biographie
Blake Richards est professeur agrégé à l'École d'informatique et au Département de neurologie et de neurochirurgie de l'Université McGill et membre du corps professoral de Mila – Institut québécois d’intelligence artificielle. Ses recherches se situent à l'intersection des neurosciences et de l'intelligence artificielle. Son laboratoire étudie les principes universels de l'intelligence qui s'appliquent aux agents naturels et artificiels. Il a reçu plusieurs distinctions pour ses travaux, notamment une bourse Arthur-B.-McDonald du Conseil de recherches en sciences naturelles et en génie du Canada (CRSNG) en 2022, le Prix du jeune chercheur de l'Association canadienne des neurosciences en 2019 et une chaire en IA Canada-CIFAR en 2018. M. Richards a en outre été titulaire d'une bourse postdoctorale Banting à l'hôpital SickKids de 2011 à 2013. Il a obtenu un doctorat en neurosciences de l'Université d'Oxford en 2010 et une licence en sciences cognitives et en IA de l'Université de Toronto en 2004.