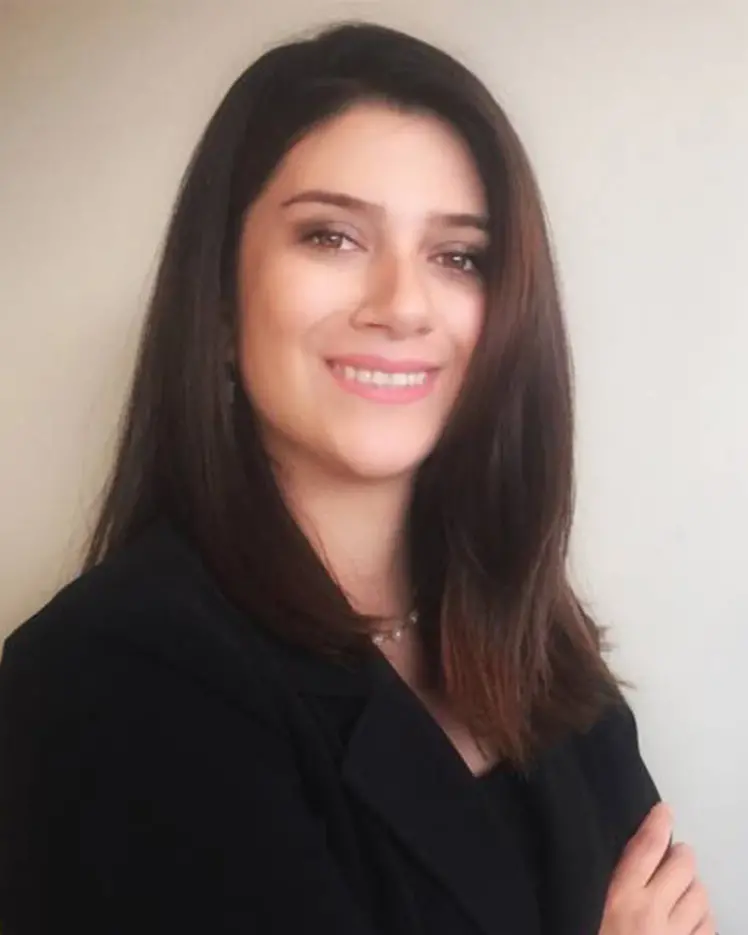
Samira Abbasgholizadeh-Rahimi
Biography
Samira Abbasgholizadeh-Rahimi (BEng, PhD) is the Canada Research Chair in Advanced Digital Primary Health Care, an assistant professor in the Department of Family Medicine at McGill University and an associate academic member at Mila – Quebec Artificial Intelligence Institute.
Rahimi is an affiliated scientist at Lady Davis Institute for Medical Research at the Jewish General Hospital, the elected president of the Canadian Operational Research Society, and director of Artificial Intelligence in Family Medicine (AIFM).
Drawing on her interdisciplinary background, her research focuses on the development and implementation of advanced digital health technologies, such as AI-enabled decision support tools, in primary health care. Her research is dedicated to enhancing the prevention and management of chronic diseases, such as cardiovascular disease, with a particular emphasis on vulnerable populations.
Rahimi‘s work as a principal investigator has been funded by the Fonds de recherche du Québec – Santé (FRQS), the Natural Sciences and Engineering Research Council (NSERC), Roche Canada, the Brocher Foundation (Switzerland), and the Strategy for Patient-Oriented Research (SPOR) of the Canadian Institutes of Health Research (CIHR).
She is the recipient of numerous awards, including the 2022 New Investigator Primary Care Research Award of North American Primary Care Research Group (NAPCRG), which recognizes exceptional contributions by emerging investigators in the field of primary care research.