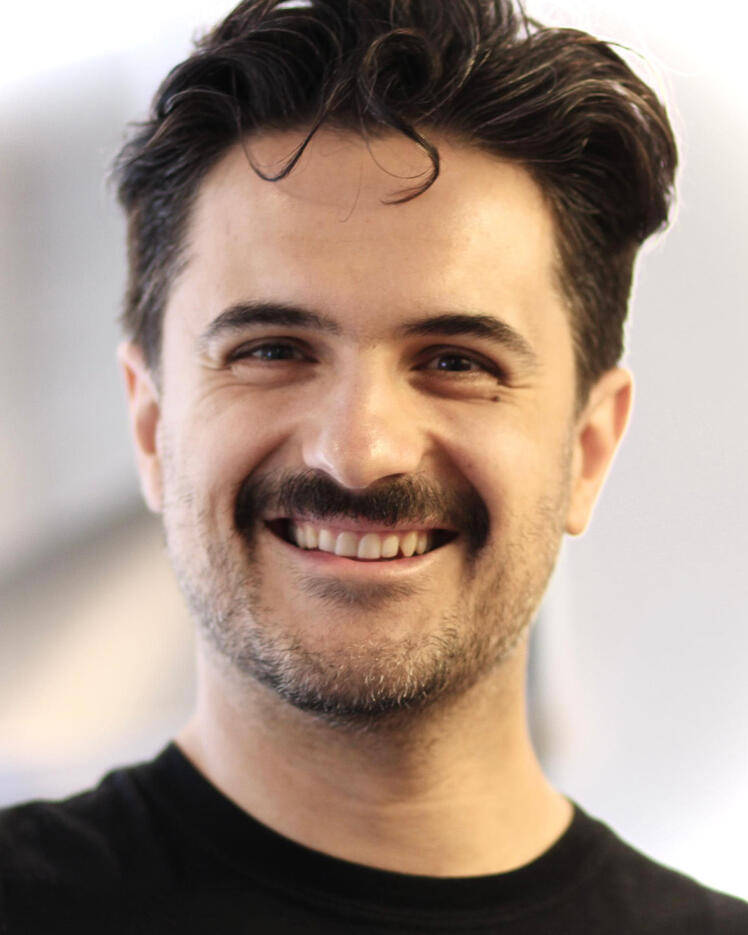
Ioannis Mitliagkas
Biography
I am an associate professor in the Department of Computer Science and Operations Research (DIRO) at Université de Montréal, as well as a core academic member of Mila – Quebec Artificial Intelligence Institute and a Canada CIFAR AI Chair. I hold a part-time position as a staff research scientist at Google DeepMind Montréal.
Previously, I was a postdoctoral scholar in the departments of statistics and computer science at Stanford University. I obtained my PhD from the Department of Electrical and Computer Engineering at the University of Texas at Austin.
My research interests lie in statistical learning and inference, with a focus on optimization, efficient large-scale and distributed algorithms, statistical learning theory and MCMC methods. My recent research has focused on methods for efficient and adaptive optimization, understanding the interaction between optimization and the dynamics of large-scale learning systems, and the dynamics of games.