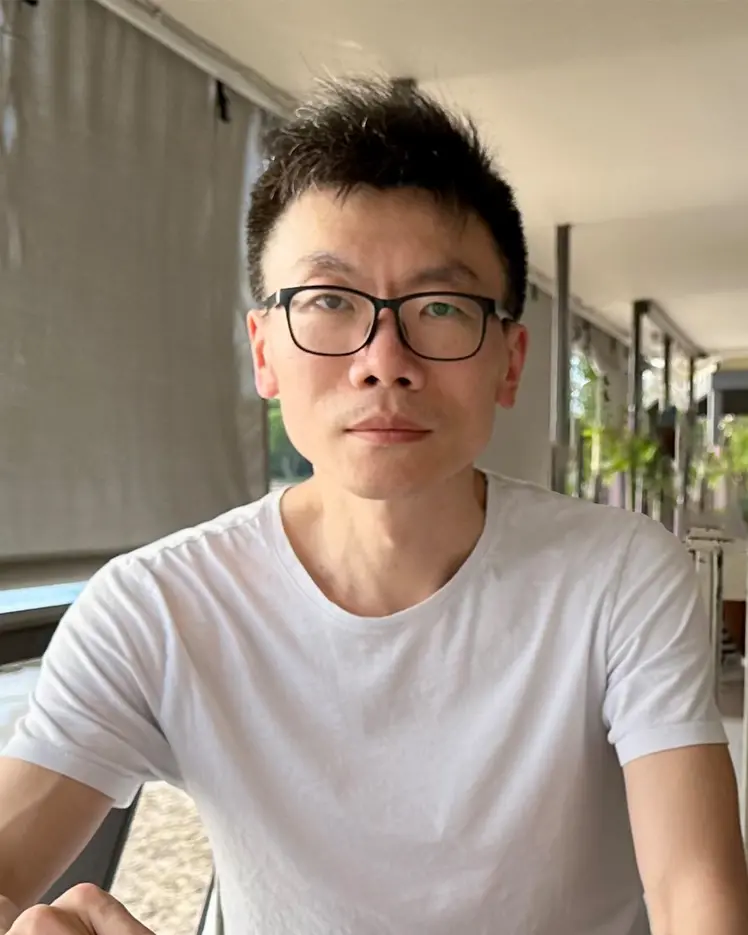
Biography
I completed my PhD degree in computer science and computational biology at the University of Toronto in 2014. Prior to joining McGill University, I was a postdoctoral associate at the Computer Science and Artificial Intelligence Laboratory (CSAIL) at MIT (2015–2018).
In general, my research program covers three main research areas that involve applied machine learning in computational genomics and health. More specifically, it focuses on developing interpretable probabilistic learning models and deep learning models to model genetic, epigenetic, electronic health record and single-cell genomic data.
By systematically integrating multimodal and longitudinal data, I aim to have impactful applications in computational medicine, including building intelligent clinical recommender systems, forecasting patient health trajectories, making personalized polygenic risk predictions, characterizing multi-trait functional genetic mutations, and dissecting cell-type-specific regulatory elements that underpin complex traits and diseases in humans.