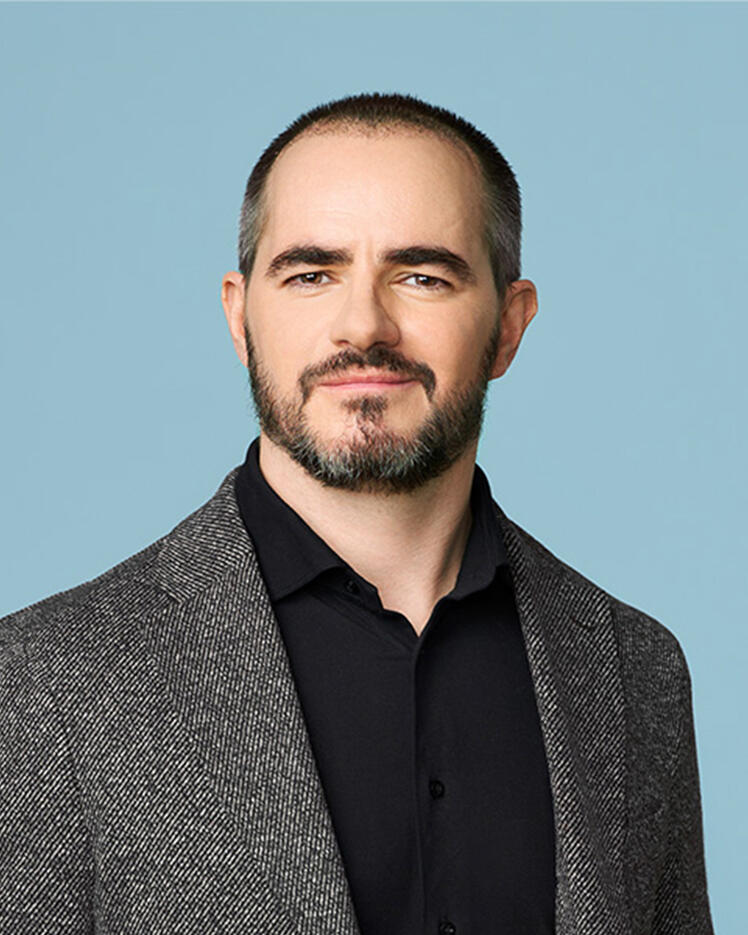
Danilo Bzdok
Biography
Danilo Bzdok is a computer scientist and medical doctor by training with a unique dual background in systems neuroscience and machine learning algorithms. After training at RWTH Aachen University (Germany), Université de Lausanne (Switzerland) and Harvard Medical School, Bzdok completed two doctoral degrees, one in neuroscience at Forschungszentrum Jülich in Germany, and another in computer science (machine learning statistics) at INRIA–Saclay and the Neurospin brain imaging centre in Paris.
Danilo is currently an associate professor at McGill University’s Faculty of Medicine and a Canada CIFAR AI Chair at Mila – Quebec Artificial Intelligence Institute. His interdisciplinary research centres around narrowing knowledge gaps in the brain basis of human-defining types of thinking in order to uncover key computational design principles underlying human intelligence.