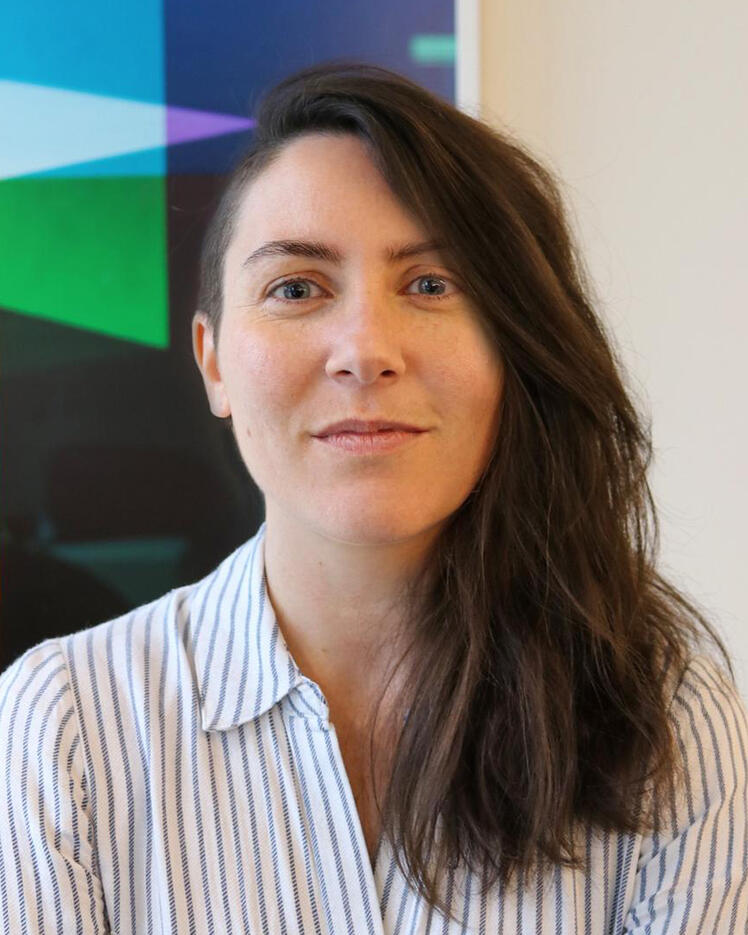
Audrey Durand
Biography
Audrey Durand is an assistant professor in the Department of Computer Science and Software Engineering and in the Department of Electrical and Computer Engineering at Université Laval.
She specializes in algorithms that learn through interaction with their environment using reinforcement learning, and is particularly interested in leveraging these approaches in health-related applications.