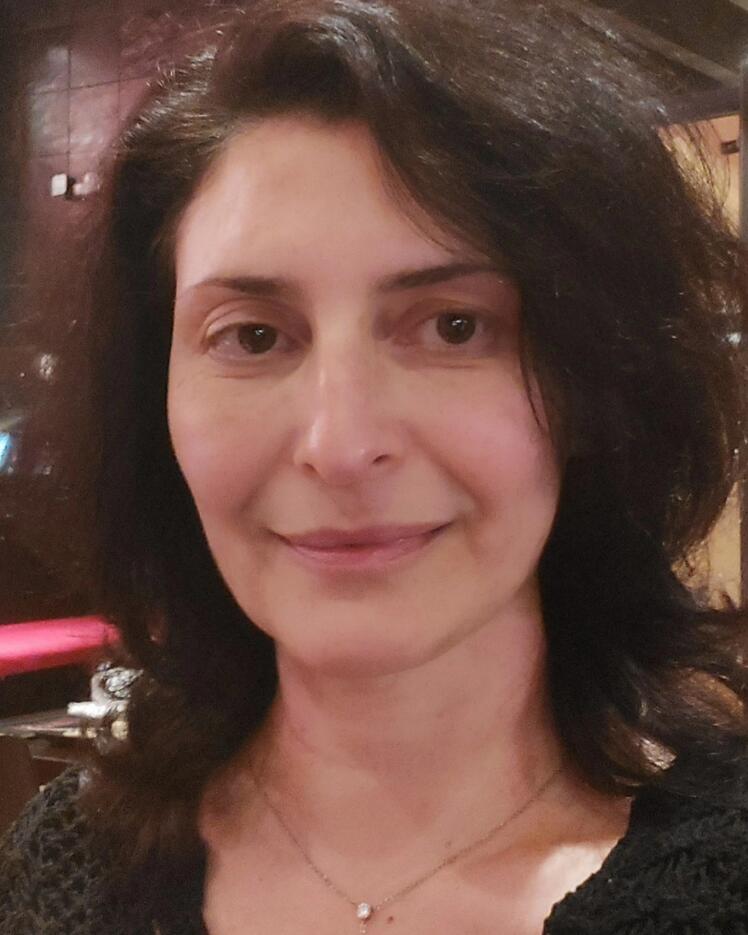
Irina Rish
Biographie
Irina Rish est professeure titulaire à l'Université de Montréal (UdeM), où elle dirige le Laboratoire d'IA autonome. Membre du corps professoral de Mila – Institut québécois d’intelligence artificielle, elle est titulaire d'une chaire d'excellence en recherche du Canada (CERC) et d'une chaire en IA Canada-CIFAR. Irina dirige le projet INCITE du ministère américain de l'Environnement au sujet des modèles de fondation évolutifs sur les superordinateurs Summit et Frontier à l'Oak Ridge Leadership Computing Facility (OLCF). Elle est cofondatrice et directrice scientifique de Nolano.ai.
Ses recherches actuelles portent sur les lois de mise à l'échelle neuronale et les comportements émergents (capacités et alignement) dans les modèles de fondation, ainsi que sur l'apprentissage continu, la généralisation hors distribution et la robustesse. Avant de se joindre à l'UdeM en 2019, Irina était chercheuse au Centre de recherche IBM Thomas J. Watson, où elle a travaillé sur divers projets à l'intersection des neurosciences et de l'IA, et dirigé le défi NeuroAI. Elle a reçu plusieurs prix IBM : ceux de l’excellence et de l’innovation exceptionnelle (2018), celui de la réalisation technique exceptionnelle (2017), et celui de l’accomplissement en recherche (2009). Elle détient 64 brevets et a écrit plus de 120 articles de recherche, plusieurs chapitres de livres, trois livres publiés et une monographie sur la modélisation éparse.