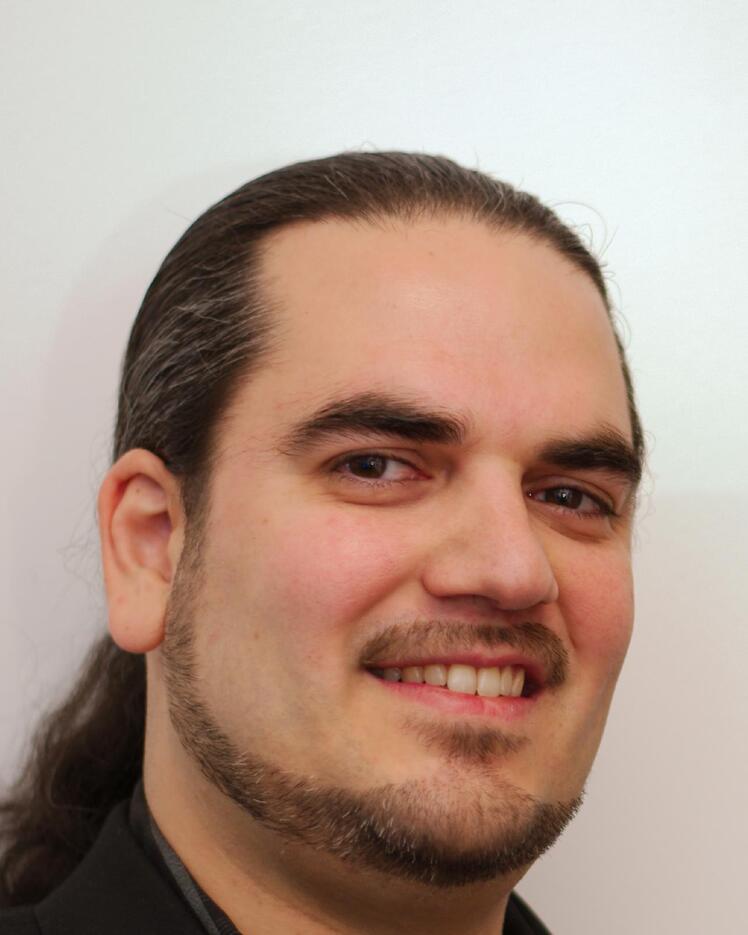
Guy Wolf
Biographie
Guy Wolf est professeur agrégé au Département de mathématiques et de statistique de l'Université de Montréal. Ses intérêts de recherche se situent au carrefour de l'apprentissage automatique, de la science des données et des mathématiques appliquées. Il s'intéresse particulièrement aux méthodes d'exploration de données qui utilisent l'apprentissage multiple et l'apprentissage géométrique profond, ainsi qu'aux applications pour l'analyse exploratoire des données biomédicales.
Ses recherches portent sur l'analyse exploratoire des données, avec des applications en bio-informatique. Ses approches sont multidisciplinaires et combinent l'apprentissage automatique, le traitement du signal et les outils mathématiques appliqués. En particulier, ses travaux récents utilisent une combinaison de géométries de diffusion et d'apprentissage profond pour trouver des modèles émergents, des dynamiques et des structures dans les mégadonnées à grande dimension (par exemple, dans la génomique et la protéomique de la cellule unique).