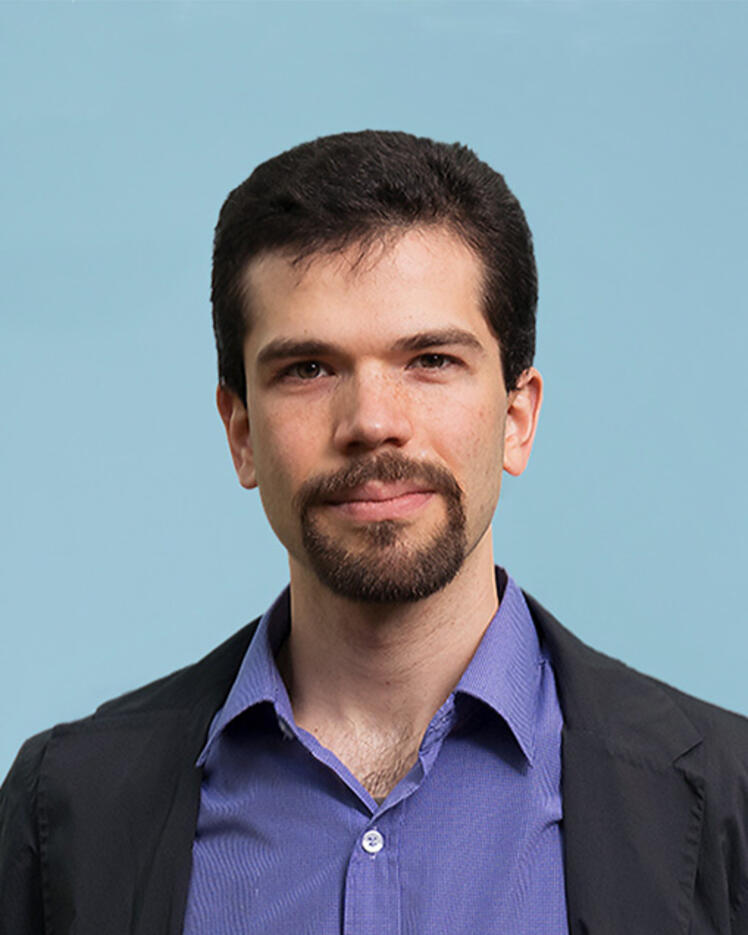
David Rolnick
Biographie
David Rolnick est professeur adjoint et titulaire d’une chaire en IA Canada-CIFAR à l'École d'informatique de l'Université McGill et membre académique principal de Mila – Institut québécois d’intelligence artificielle. Ses travaux portent sur les applications de l'apprentissage automatique dans la lutte contre le changement climatique. Il est cofondateur et président de Climate Change AI et codirecteur scientifique de Sustainability in the Digital Age. David Rolnick a obtenu un doctorat en mathématiques appliquées du Massachusetts Institute of Technology (MIT). Il a été chercheur postdoctoral en sciences mathématiques à la National Science Foundation (NSF), chercheur diplômé à la NSF et boursier Fulbright. Il a figuré sur la liste des « 35 innovateurs de moins de 35 ans » de la MIT Technology Review en 2021.