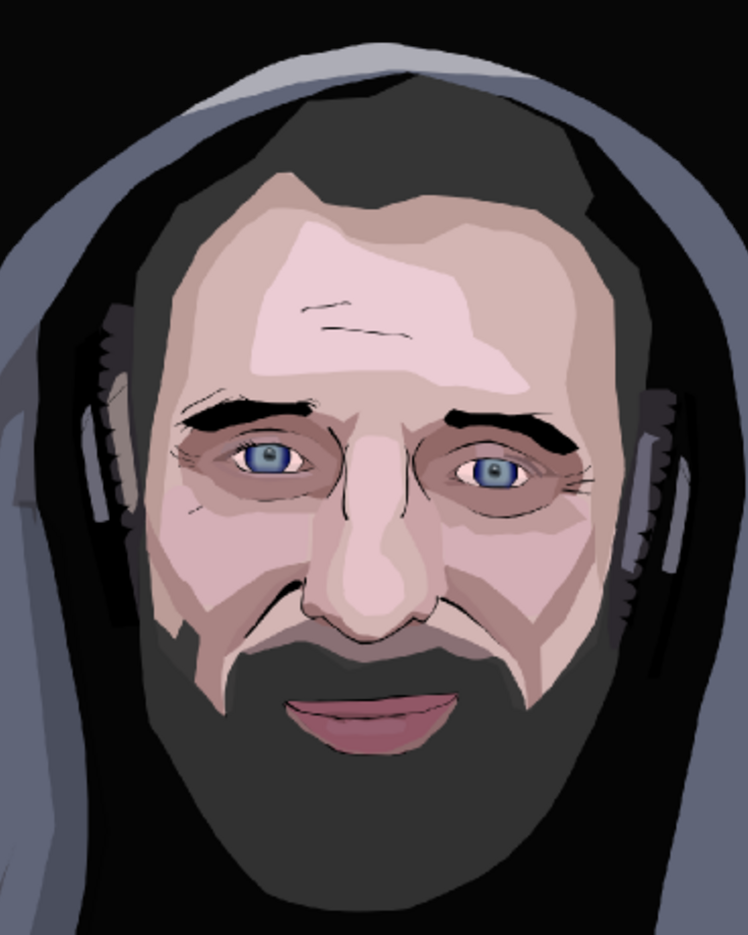
Pierre (Louis) Bellec
Biography
I am an associate professor in the Department of Psychology at Université de Montréal, and a principal investigator at SIMEXP – Laboratory for Brain Simulation and Exploration at the CRIUGM (research centre of the Montréal university geriatrics institute). I recently joined Mila – Quebec Artificial Intelligence Institute as an affiliate member, and I also supervise students in computer science (cognitive computational neuroscience) at the Department of Computer Science and Operations Research (DIRO), Université de Montréal.
My main research interest is training artificial neural networks to jointly mimic individual human brain activity and behaviour. To achieve this goal, I lead an intensive effort in individual data collection in neuroimaging (fMRI, MEG) and the Courtois project on neuronal modelling (CNeuroMod). I am a senior Fonds de recherche du Québec - Santé (FRQS) scholar, a member of UNIQUE (Quebec alliance for unifying neuroscience and AI), and the scientific director of the CRIUGM’s functional neuroimaging unit.