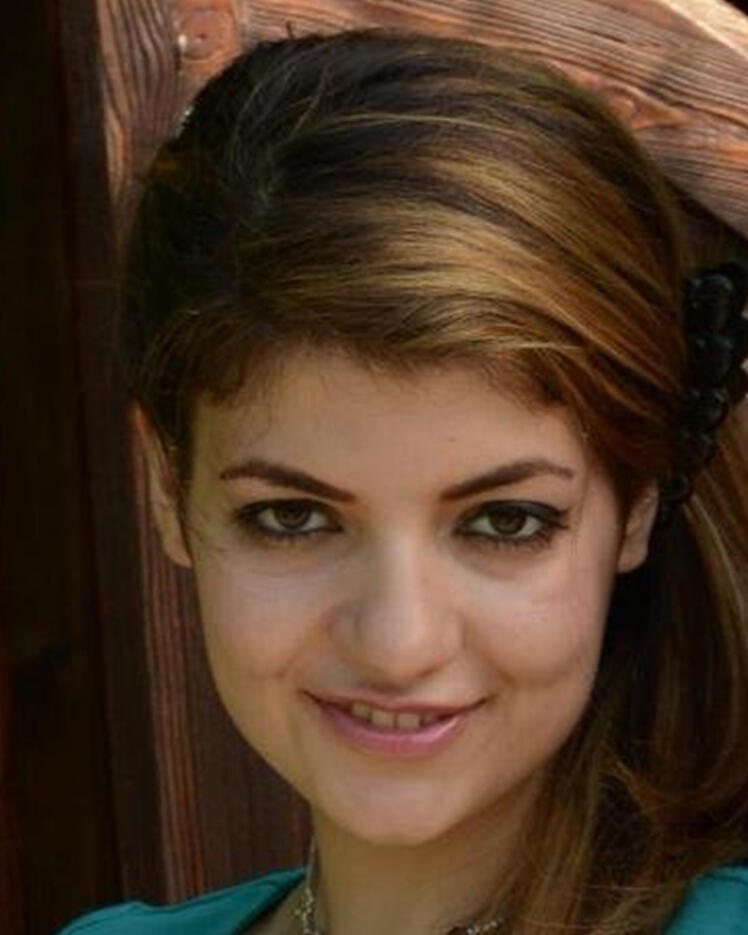
Negar Rostamzadeh
Biography
Negar Rostamzadeh is a Senior Research Scientist at Google Responsible AI team and an Associate Industrial member at Mila - Quebec Artificial Intelligence Institute. Her research primarily focuses on understanding the social implications of machine learning and evaluation systems, as well as developing equitable and fair ML systems.
Negar holds a deep interest in the creative applications of computer vision and their impact on society and artists. She is the founder and program chair of the workshop series, "Computer Vision for Fashion, Art, and Design," as well as "Ethical Considerations in Creative Applications," featured at Computer Vision venues from ECCV 2018 to CVPR 2023.
Before joining Google, Negar worked as a research scientist at Element AI (Service Now), where she specialized in efficient learning from limited data in computer vision and multi-modal problems.
She completed her PhD in 2017 at the University of Trento under the supervision of Prof. Nicu Sebe, focusing on Video Understanding problems. She also spent two years at MILA (2015-2017), working on attention mechanisms in videos, generative models, and video captioning under the guidance of Prof. Aaron Courville. In 2016, she had the opportunity to intern with Google's Machine Intelligence team.
Negar actively contributes to various community engagements within the AI community. She has served as the program chair for the workshop series, "Science meets Engineering of Deep Learning," at ICLR, FAccT, and NeurIPS. Since 2020, she has been a board member of the Montreal AI Symposium, and in 2019, she held the position of Senior Program Chair. Negar is also an Area Chair for Vision Conferences such as CVPR and ICCV, and gave multiple keynotes in various workshops and conferences.