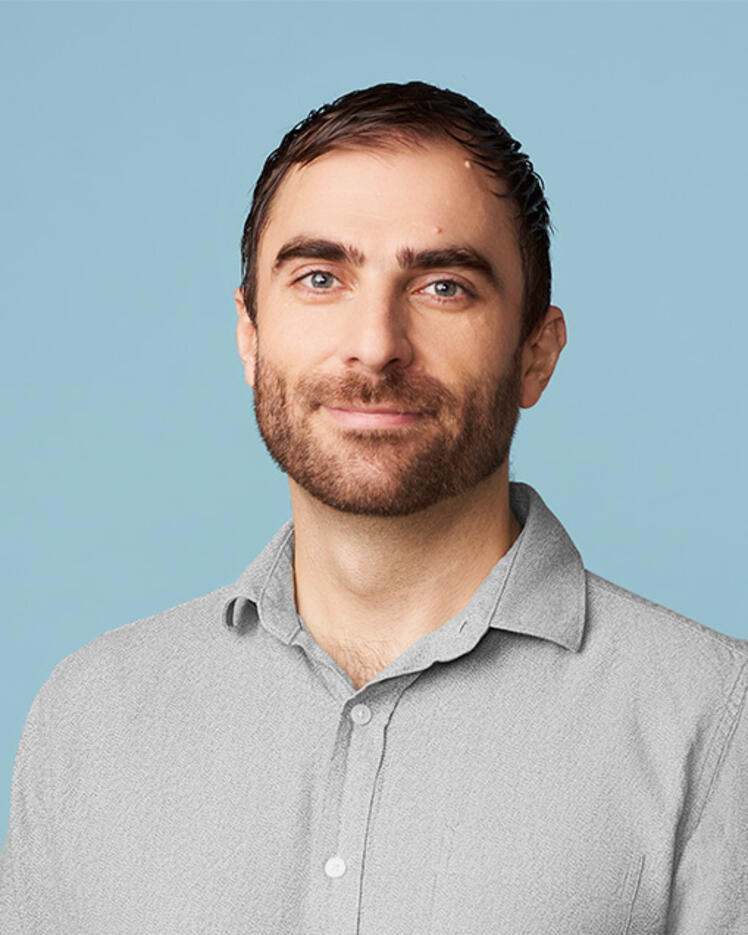
Glen Berseth
Biography
Glen Berseth is an assistant professor in the Department of Computer Science and Operations Research (DIRO) at Université de Montréal and a core academic member of Mila – Quebec Artificial Intelligence Institute.
He is a Canada CIFAR AI Chair and co-directs the Robotics and Embodied AI Lab (REAL). He was formerly a postdoctoral researcher at Berkeley Artificial Intelligence Research (BAIR), working with Sergey Levine.
Berseth’s previous and current research has focused on solving sequential decision-making problems (planning) for real-world autonomous learning systems (robots). More specifically, his research has focused on human-robot collaboration, reinforcement, and continual-, meta-, multi-agent and hierarchical learning.
He has published in the top venues in robotics, machine learning and computer animation. He teaches a course on robot learning at Université de Montréal and at Mila, in which he covers the most recent research on machine learning techniques for creating generalist robots.