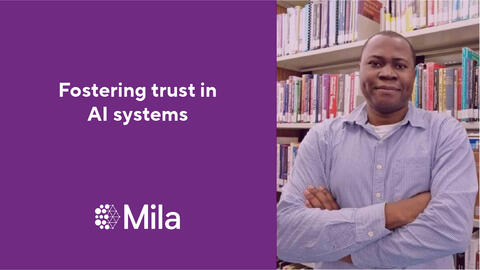
Artificial intelligence-based systems contain biases that disproportionately affect populations underrepresented in the data used to train them. On the occasion of Black History Month, Mila researcher Foutse Khomh, a professor at Polytechnique Montreal and Canada CIFAR AI Chair, explains how he takes these biases into account in his research and discusses solutions to address them.
Foutse Khomh, a professor at Polytechnique Montréal for the past ten years, has been working on numerous projects including satellite image processing scrutinizing methane emissions in the atmosphere, robots circulating in production lines to anticipate maintenance needs and modeling tools for the aerospace industry.
He is particularly interested in questions about trust in AI systems to ensure they are safe, reliable and do not have adverse effects on users, especially on disadvantaged segments of society who may not have been sufficiently taken into account throughout the development of these systems.
For several years, his research group has been teaming up with ethics specialists to better address various social issues raised by the software they develop and to bring up and correct any problems, particularly those related to bias.
"Bias issues are at the heart of AI technologies because deep learning–in particular–depends heavily on the data used to extract functionalities and properties. The information encoded in the data is going to hugely influence what the resulting system can do, so we need to make sure that different facets of our society are well represented in this information so that this system is also tailored to the diversity of society," according to Foutse Khomh.
"The data is unbalanced because historically, certain parts of society like Black and First Nations people have been less represented in the data that we use to train the models. And even when there is representation, it is not always adequate because sometimes it is tainted by our existing biases."
This lack of representation induces bias in the data feeding the models and further marginalizes already underrepresented populations. For example, some facial recognition systems have a very high error rate for people with dark skin.
These models were trained with a disparity in the representation of different skin colors and thus have fewer points of comparison to properly identify darker-skinned people, leading to discrimination in the real world.
"Since the algorithm learns the recurring structures embedded in the data, the lack of representativeness is therefore one of the main problems. A lot of the solution comes from being aware of this state of imbalance in the data. Through technology, we can make fixes, but then being able to provide evidence that those fixes were effective is crucial to controlling the behavior of the system."
One solution his research group has applied to counter the biases inherent in AI-based systems is to implement evaluation mechanisms throughout the software's lifecycle to detect potential problems in its operation and then fix or replace it with a more reliable version.
"We can address the shortcomings of a model if we have a good understanding of where they come from and how they appear. These models will never be perfect, but if we understand enough about how they work, we can build a robust system.”
According to Foutse Khomh, a more diverse education and awareness of bias issues would also lead to a better representation of social realities in the implementation of AI-based systems.
"There is a need for the public to understand how these technologies are developed and the impact they can have on them. There's a need for education and it's through that interaction that we're going to improve the quality of the data we're using and improve the distribution of skills to work on these technologies and make sure that we're developing technologies that don't just benefit the few but the many in society."
Foutse Khomh points out that Mila researchers are fairly well aware of the issue, thanks in part to initiatives such as the International summer school on bias and discrimination in AI, but that more progress remains to be made.
"In the research that we do, we should build that in, it should not be a problem we only care about after we build a system, but a problem we are aware of throughout the process of ideating and building these systems. We also need to make sure that we diversify the pool of people who are looking for solutions to these problems to get more unique ideas, approach the problem in the right way and collectively come up with answers."