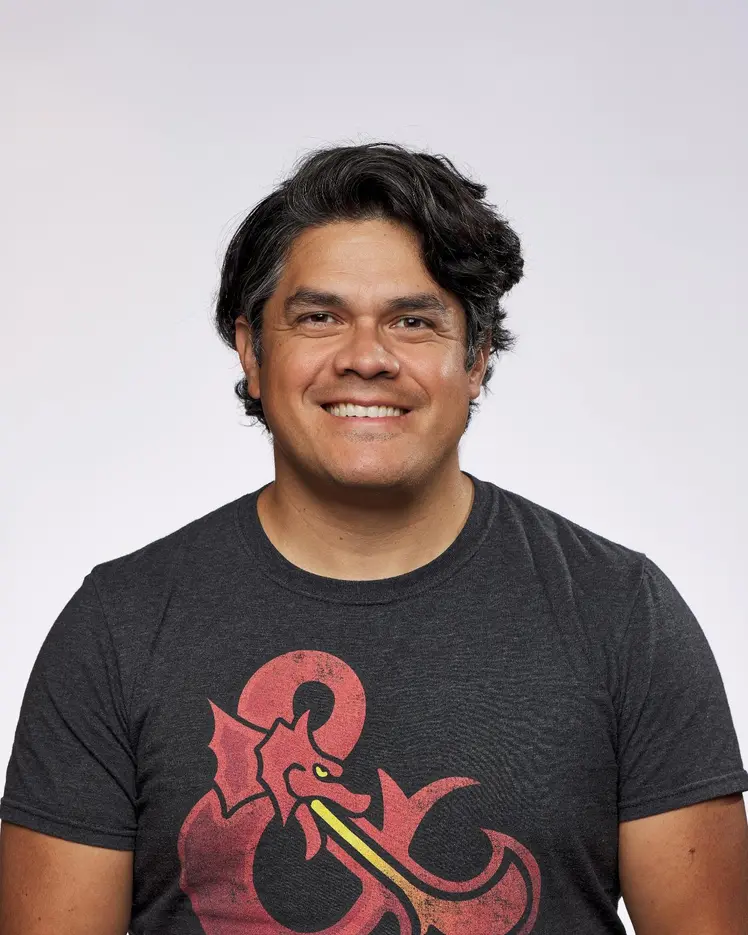
Pablo Samuel Castro
Biography
Pablo Samuel Castro was born and raised in Quito, Ecuador, and moved to Montréal after high school to study at McGill University. For his PhD, he studied reinforcement learning with Doina Precup and Prakash Panangaden at McGill. Castro has been working at Google for over eleven years. He is currently a staff research scientist at Google DeepMind in Montreal, where he conducts fundamental reinforcement learning research and is a regular advocate for increasing LatinX representation in the research community.
He is also an adjunct professor in the Department of Computer Science and Operations Research (DIRO) at Université de Montréal. In addition to his interest in coding, AI and math, Castro is an active musician.