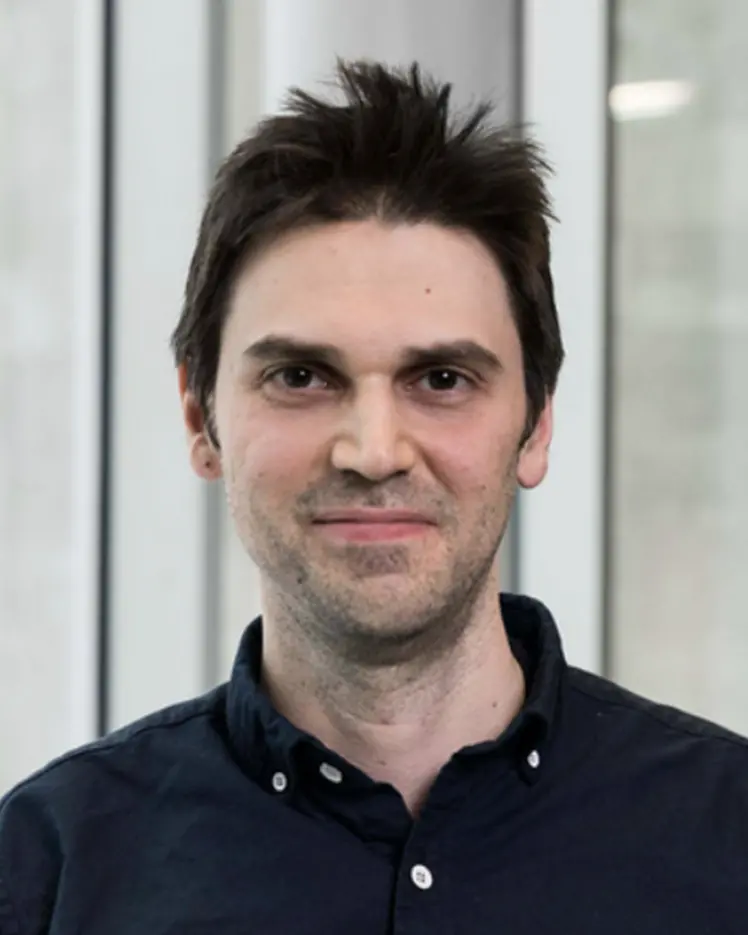
Cem (Yusuf) Subakan
Biography
Cem Subakan is an assistant professor in the Computer Science and Software Engineering Department at Université Laval, and an affiliate assistant professor in the Computer Science and Software Engineering Department at Concordia University. He is also an associate academic member of Mila – Quebec Artificial Intelligence Institute. After receiving his PhD in computer science from the University of Illinois at Urbana-Champaign (UIUC), Subakan did a postdoc at Mila. He serves as a reviewer for many conferences including NeurIPS, ICML, ICLR, ICASSP and MLSP, as well as for journals, such as IEEE Signal Processing Letters and IEEE Transactions on Audio, Speech, and Language Processing. His principal research interest is machine learning for speech and audio. More specifically, he works on deep learning for source separation and speech enhancement under realistic conditions, neural network interpretability, continual learning and multi-modal learning.
Subakan was awarded the Best Student Paper Award at the 2017 IEEE Machine Learning for Signal Processing Conference, and also obtained a Sabura Muroga Fellowship from UIUC’s Department of Computer Science. He is a core contributor to the SpeechBrain project, leading the speech separation component.