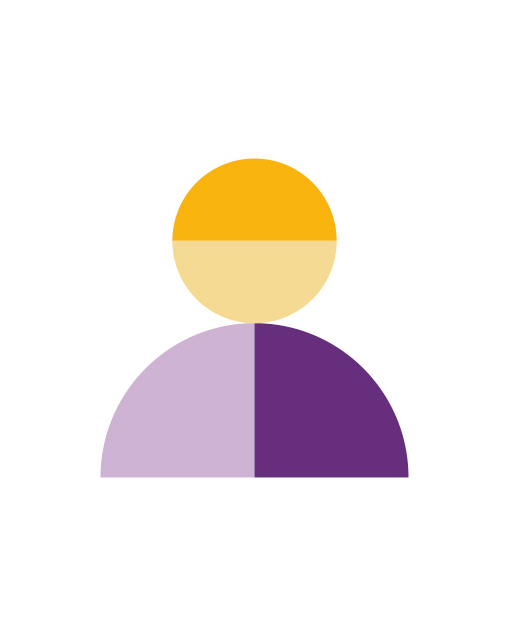
David Scott Krueger
Biography
David Krueger is an Assistant Professor in Robust, Reasoning and Responsible AI in the Department of Computer Science and Operations Research (DIRO) at University of Montreal, and a Core Academic Member at Mila - Quebec Artificial Intelligence Institute, UC Berkeley's Center for Human-Compatible AI (CHAI), and the Center for the Study of Existential Risk (CSER). His work focuses on reducing the risk of human extinction from artificial intelligence (AI x-risk) through technical research as well as education, outreach, governance and advocacy.
His research spans many areas of Deep Learning, AI Alignment, AI Safety and AI Ethics, including alignment failure modes, algorithmic manipulation, interpretability, robustness, and understanding how AI systems learn and generalize. He has been featured in media outlets including ITV's Good Morning Britain, Al Jazeera's Inside Story, France 24, New Scientist and the Associated Press.
David completed his graduate studies at the University of Montreal and Mila - Quebec Artificial Intelligence Institute, working with Yoshua Bengio, Roland Memisevic, and Aaron Courville.