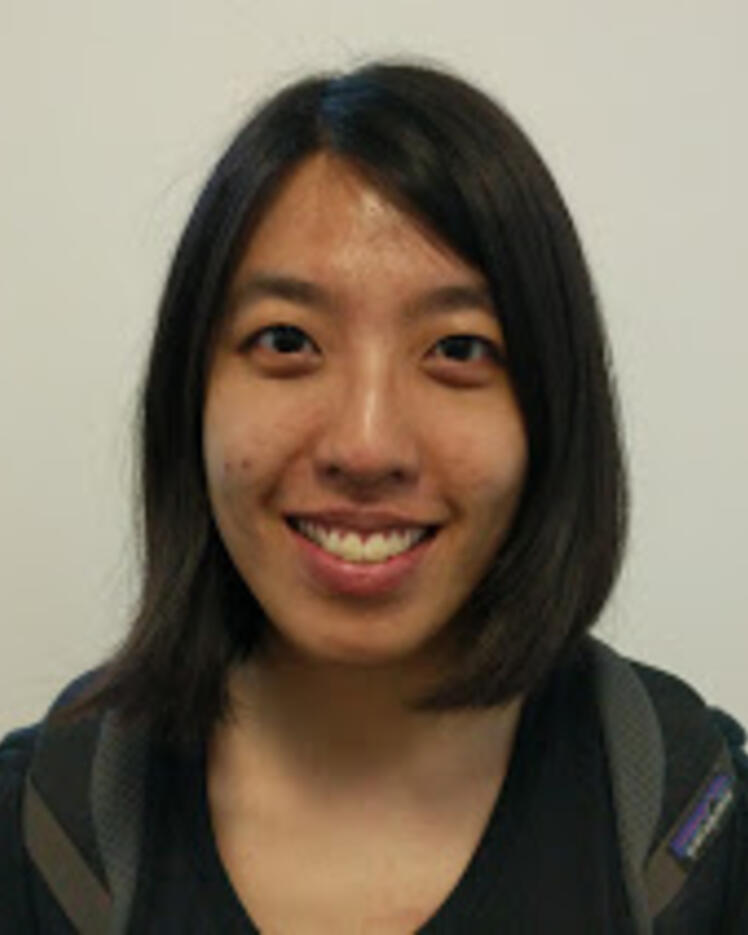
Anna (Cheng-Zhi) Huang
Biography
Anna Huang is a research scientist at Google DeepMind, where she works on the Magenta Project. She is also an adjunct professor at Université de Montréal. Her research focuses on designing generative models and interfaces to support music making and, more generally, the creative process. Her work lies at the intersection of machine learning, human-computer interaction and music.
Huang is the creator of Music Transformer and Coconet, the ML model that powered Google’s first AI Doodle, the Bach Doodle, which in two days harmonized 55 million melodies from users around the world. She has been an organizer and judge for the international AI Song Contest for the past few years, and was a guest editor for TISMIR's special issue on AI and musical creativity.
Huang has a PhD from Harvard University, an MSc from the MIT Media Lab, and a dual bachelor's degree in computer science and music composition from the University of Southern California.