
The world as we know it runs on electricity, and power lines are a critical infrastructure for delivering power to homes and businesses. To ensure their reliable operation, they must be routinely inspected for issues and anomalies, but manually inspecting the extensive networks requires significant time, resources, and manpower. For several years, Hydro-Québec, a Mila partner organization, has worked to integrate robotics in power grid inspections, developing a fleet of robots to do the dangerous, dizzying task of skimming power lines hundreds of feet in the air.
Yet across the thousands of kilometers of power lines in Quebec, the odds of finding an anomaly — a scratch, a stain, a broken wire, or even just some bird poop — are deceptively rare. For that reason, the vast amount of collected data poses a significant analytical challenge, since the anomalies being identified are so uncommon. This question led to an applied research project between Mila and Hydro-Québec to help improve power line maintenance by efficiently identifying anomalies using AI.
A comprehensive approach
As part of a years-long partnership between Mila and Hydro-Québec, Mila’s applied researchers Akshatha Arodi Nagaraja, Margaux Luck, Ge Li, Aldo Zaimi, and Gaétan Marceau Caron launched the applied research project with experts at Hydro-Québec. Their objectives were to produce a robust dataset of annotated anomalies that could enable more extensive and in-depth algorithm analysis for inspecting cable conditions across the network, and to adapt these algorithms for few-shot and zero-shot learning scenarios.
In order for the data to reflect the diverse anomalies encountered on power line cables, Mila researchers collaborated with Hydro-Québec experts to create CableInspect-AD, a high-quality, publicly available dataset for model training and evaluation tailored for power line inspections. Curated by Hydro-Québec’s research institute, IREQ, this unique dataset includes a wide range of real-world anomalies, such as scratches and broken strands, presented through thousands of high-resolution images of three distinct power line cable types showcasing seven common defects at varying severity levels.
With this robust dataset in hand, the Mila team explored different AI methods for anomaly detection. They prioritized those that required minimal training data and no separate validation set, in keeping with real-world constraints where extensive training data is often impractical. Ultimately, the team attempted zero-shot learning with Vision-Learning Models (VLMs), which can detect anomalies without prior training on specific defects. Surprisingly, these models performed very well, identifying anomalies without explicit training on power line defects, and even outperforming traditional methods in some cases.
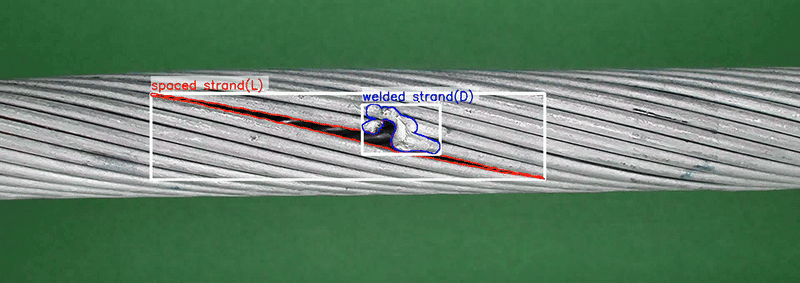
An impactful collaboration
The project, which produced a paper accepted at NeurIPS 2024, is expected to enable faster, more effective anomaly detection, allowing Hydro-Québec to improve the efficiency of their inspections and the resilience of their power grids. The team expressed their satisfaction at being able to publish and showcase their findings at an international conference such as NeurIPS.
“Getting this dataset accepted at a conference was very rewarding to us and our collaborators at Hydro-Québec, especially because we were able to raise awareness of the community to this very important real-world problem in the industry” said Akshatha Arodi Nagaraja, Senior Applied Research Scientist at Mila and joint first author of the paper along with Margaux Luck.
Committed to an open science approach, the team also released the dataset and code under a non-commercial open-source license, to allow training and evaluation of AI models across the research community, and to help accelerate progress.
Thanks to Mila’s applied research team, this applied research project fills a critical gap by offering a realistic benchmark for AI models, and by efficiently monitoring infrastructure conditions and facilitating maintenance. This not only helps create a more resilient power grid, but also underscores the strengths of Mila's collaborative research approach to address specific domain needs, combining the expertise of AI researchers with the domain knowledge of industry experts. Pleased with the applied research’s success, Hydro-Québec and Mila look forward to collaborating in the future, expanding their partnership to new, energizing projects.