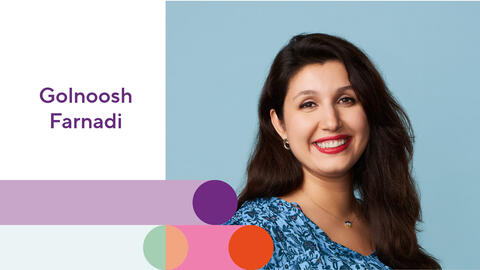
Golnoosh Farnadi was born in Iran and earned a PhD in Belgium by designing a model to predict personality traits based on people’s digital footprint including images, texts, relationships and interactions on social media. When recruitment companies started reaching out to use her tools and hire people based on the arbitrary decision of a computer program, she noticed how her work could be misused and shifted her focus to algorithmic biases. To mark International Women’s Day, Mila highlights her quest to make AI systems fairer and less biased against marginalized groups.
Now a researcher at Mila, professor at HEC Montréal and University of Montreal and holding a Canada CIFAR AI Chair, she has been working since 2017 on the trustworthiness of machine learning systems through studying aspects of algorithmic discrimination, fairness and privacy.
Real-world impacts of biases
Projects like Gender Shades revealed that women with darker skins were more likely to be misidentified by facial recognition software because the datasets used to train them mostly included faces of white men.
“The model could not recognize them because they focus on patterns they most often encounter and learn from these patterns.”
However, even if datasets are fixed to ensure a fair representation of the population, questions still remain on whether tools like facial recognition should at all be used, and by whom.
Any machine learning system contains errors due to generalization –a way for AI systems to extrapolate from their previous experiences to work with unseen data– which could lead to real-world harm because it does not take into account that the distribution of data, like crime rate for a specific neighborhood, can change over time.
Biases were found in recruitment tools not showing highly-paid or technical jobs to women, in loan approvals denying requests from Black applicants or in wrongful risk assessment regarding criminal activity.
“While addressing data issues or algorithmic bias can improve the fairness of a machine learning model, it may not fully mitigate the potential harm caused by deploying the model in a high-stakes decision-making system,” Dr. Farnadi warned.
“Using AI and machine learning models should only be used as a helper or observer but should not be used as a decision-making tool without supervision.”
Laws to ensure fairness – e.g. against discrimination based on race, gender or sexual orientation – do not automatically translate into mathematical formulations within AI systems. Moreover, Golnoosh Farnadi says efforts to tackle algorithmic biases should be focusing on areas where these laws already exist.
Where biases come from
Biases can appear anywhere throughout the pipeline of AI systems development: in the data used to train the model, in the model itself, and in the outcome of the model.
They can emerge from datasets that are unbalanced or historically discriminatory. For instance, women and people of color have a historically lower applying and approval rate for bank loans, which can impact predictions of models based on such data and could lead to further discrimination.
“Training the model with this data will also lead it to contain biases, and resulting models may even amplify such biases,” Dr. Farnadi explained.
Bias in the outcome can arise from people using biased AI systems for decision-making without fully understanding how the model works, blindly trusting the model and making judgements based on its conclusions.
“Simply correcting bias in the model does not guarantee that discrimination in the decision-making process will also be resolved. Therefore, we must exercise caution in our utilization of machine learning models.”
Tackling biases
Defining fairness is complex because of the various perspectives to consider, and addressing biases within AI systems can be challenging because groups are often not homogeneous – e.g. men/women – and individuals within these groups have different profiles – e.g. black men/black women.
“When you’re at a group level, you’re defining that all women are similar and put them in one group and try to equalize their chances or correct the errors of the system to make the group equal to a group of men. But when you go down to the individual level, you have to define what is the measure of similarity between two individuals, and then it is highly contextual. Two applicants similar in terms of qualifications for a job will be very different from the similarity of two patients in healthcare.”
Fixing the data for a certain domain can be an impossible task, however, and historical disparities mean that some data just doesn’t exist. Instead, Golnoosh Farnadi develops novel algorithmic designs that take into account fairness, robustness and privacy concerns. This is especially timely as increasingly powerful models require sensitive data to generate accurate predictions.
She is also increasingly interested in the ethics of generative AI models like ChatGPT.
“As humans, we like to trust, and the problem in AI and machine learning models is that we trust these models, but they should not be trusted because they don’t give out any sources and as a user, you cannot know whether you are being discriminated against. The information one user gets is different from what another user gets, and we run the risk of living in AI-created bubbles as use of these models increases.”
Dr. Farnadi decries that most data used to train modern models come from North America and means that other parts of the world risk being underrepresented.
“We have to break the trend of bigger and bigger models because it creates a monopoly structure leading to more biases because it works for one community in particular,” Golnoosh Farnadi concluded.
Working with local data in a more democratic and less centralized way, would also help reduce biases.
“This would also give smaller players with less access to resources a chance to contribute.”