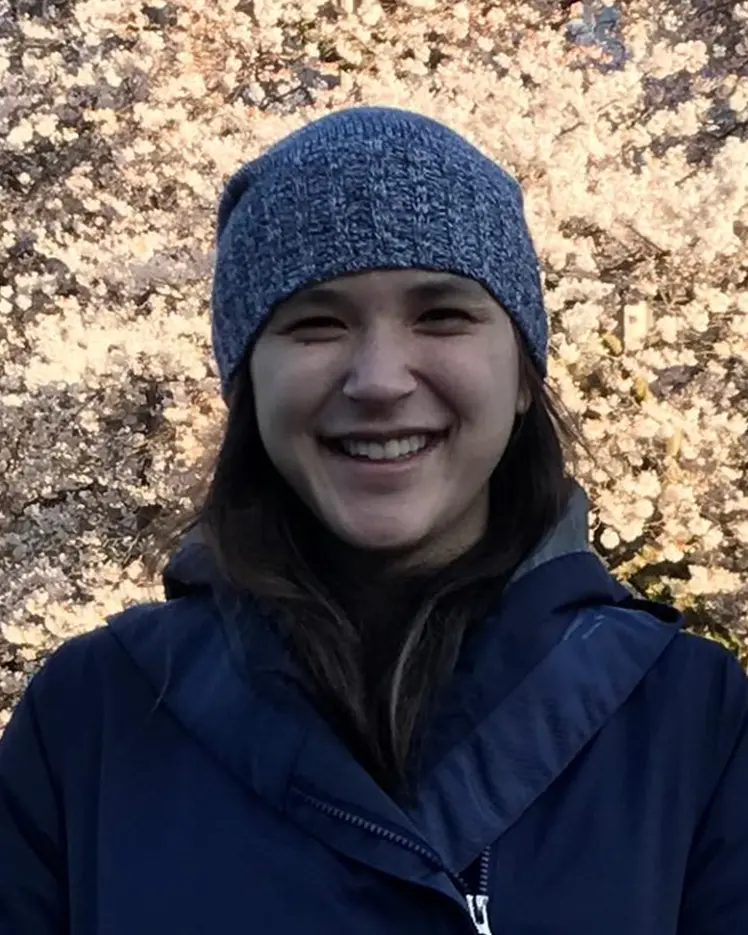
Courtney Paquette
Biography
Courtney Paquette is an assistant professor at McGill University and a Canada CIFAR AI Chair at Mila – Quebec Artificial Intelligence Institute.
Her research focuses on designing and analyzing algorithms for large-scale optimization problems, motivated by applications in data science.
She received her PhD in mathematics from the University of Washington (2017), held postdoctoral positions at Lehigh University (2017–2018) and the University of Waterloo (NSF postdoctoral fellowship, 2018–2019), and was a research scientist at Google Brain in Montréal (2019–2020).