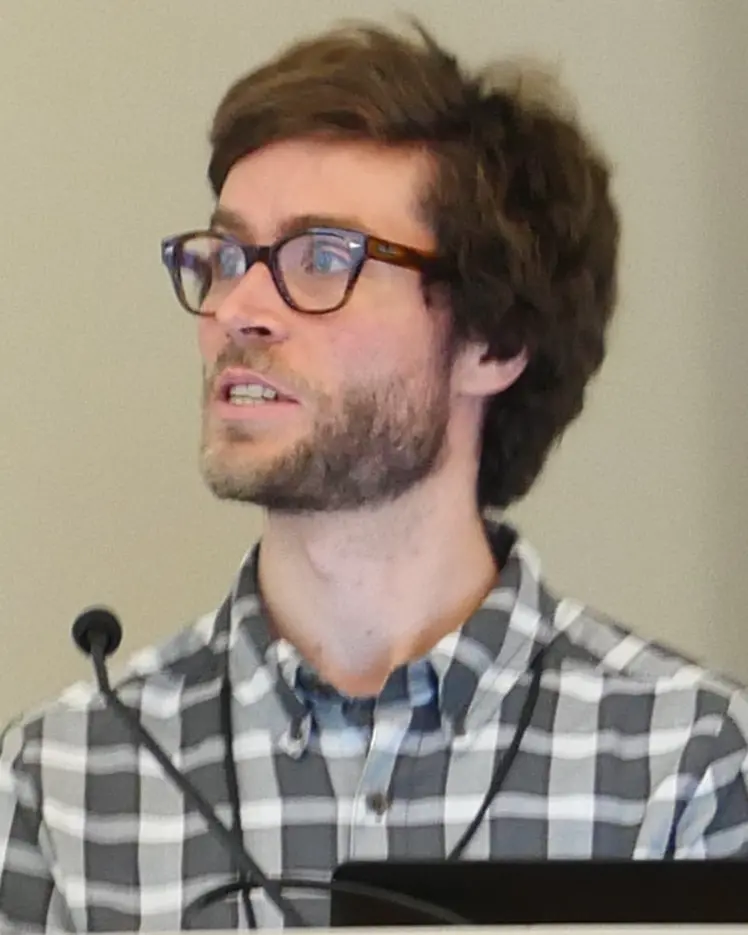
Antoine Lesage-Landry
Biography
I am an Associate professor in the Department of Electrical Engineering at Polytechnique Montréal. I received my BEng degree in engineering physics from Polytechnique Montréal in 2015, and my PhD degree in electrical engineering from the University of Toronto in 2019. I was a postdoctoral scholar in the Energy & Resources Group at the University of California, Berkeley, from 2019 to 2020. My research interests include optimization, online learning and machine learning, and their application to power systems with renewable generation.