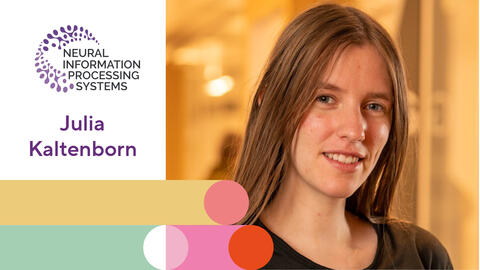
Julia Kaltenborn, a PhD student at Mila, saw her paper ClimateSet: A Large-Scale Climate Model Dataset for Machine Learning accepted at NeurIPS 2023. The research aims to provide the machine learning community with a dataset encapsulating common climate models to help speed up and improve long-term climate projections.
Julia Kaltenborn has a background in cognitive sciences and started doing research at Mila at the intersection of machine learning, her technical expertise, and climate science, her personal passion. She has been involved in climate activism groups for years and has taken part in the Juneau Icefield Research Program this year.
“I'm both invested in doing hands-on fieldwork to understand the data that we are working with and doing machine learning development in order to be able to predict and analyze the patterns that we can observe,” she explained.
AI to advance climate science
One of the key hurdles of today’s climate science is that running simulations is very time-consuming and compute-intensive. Julia Kaltenborn’s research work aims to make climate projections over a long period of time better and easier with machine learning.
The various models available to the climate science community today include uncertainty, meaning two different climate models give two different predictions, thus complicating the work of researchers and policymakers wanting to prepare for the climate crisis.
For the machine learning community, current research on climate projection is made difficult by the limited access to large scale, robust and consistent climate data, Julia Kaltenborn noted.
ClimateSet, created by a multidisciplinary team, aims to facilitate more accurate and localized projections than what is currently possible by including inputs and outputs of 36 climate models from around the world.
With this paper, “we tried to get a really large and consistent data set that can be used by machine learning scientists” to make long-term climate projections, Julia Kaltenborn explained.
It can be used to retrieve different climate scenarios, get very specific climate model data, or build large foundational climate models.
“I honestly hope that it provides the data that our community needs in order to use machine learning to answer more climate-centered questions,” Julia Kaltenborn said.
Helping decision-makers
Charlotte Lange, second author of the paper, said that ClimateSet aims to facilitate the use of AI to respond to the climate crisis.
“Climate model data is something like the dream playground and ultimate boss for machine learning developers due to its vast dimensionality, complexity, and impact,” she said.
“However, getting there so far meant navigating through a worldwide maze of inconsistencies, global distribution and climate jargon. ClimateSet aims to be the shortcut for the machine learning community - or in other words, a quick way to level up in preparing for the climate crisis,” she added.
The dataset was elaborated in collaboration with Intel.
Yaniv Gurwicz, research scientist at Intel Labs, said that “we aim to speed up climate projections by orders of magnitude to make them more accessible and practical for the machine learning and climate science research communities, and to enable climate projections for new emission scenarios not previously considered.
“Open-sourcing ClimateSet, the largest hub of climate models, is a major step towards achieving fast climate change predictions and will contribute to addressing meaningful climate-related tasks,” he added.
“Predicting climate change for the decades to come is a fundamental necessity for decision-makers to adopt the right climate policy,” he emphasized.
Eventually, making climate data more available and accessible to the machine learning community can result in new applications and findings that will support better decision-making at the political level, Julia Kaltenborn said.
“Machine learning can help emulate more climate change scenarios to help policymakers analyze a wider range of outcomes and focus on very specific, regional data to better understand and prepare for the climate crisis,” she concluded.