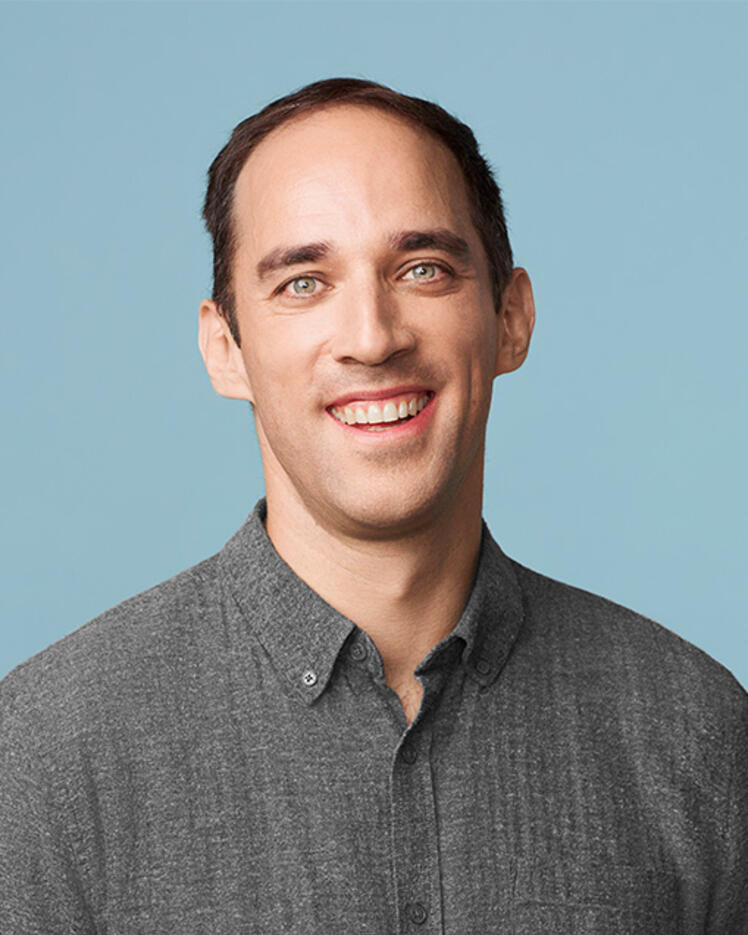
Laurent Charlin
Biographie
Laurent Charlin est titulaire d’une chaire en IA Canada-CIFAR à Mila – Institut québécois d’intelligence artificielle et professeur associé à HEC Montréal. Il est également membre principal à Mila. Ses recherches portent sur le développement de nouveaux modèles d'apprentissage automatique pour aider à la prise de décision. Ses travaux récents concernent l'apprentissage à partir de données qui évoluent dans le temps. Il travaille également sur des applications dans des domaines tels que les systèmes de recommandation et l'optimisation. Il est l'auteur de publications très citées sur les systèmes de dialogue (chatbots). Laurent Charlin a codéveloppé le Toronto Paper Matching System (TPMS), qui a été largement utilisé dans les conférences d'informatique pour faire correspondre les réviseur·euse·s aux articles. Il a également contribué à plusieurs MOOC récents, et a donné des conférences d'introduction et des interviews dans les médias pour contribuer au transfert de connaissances et améliorer la culture de l'IA.