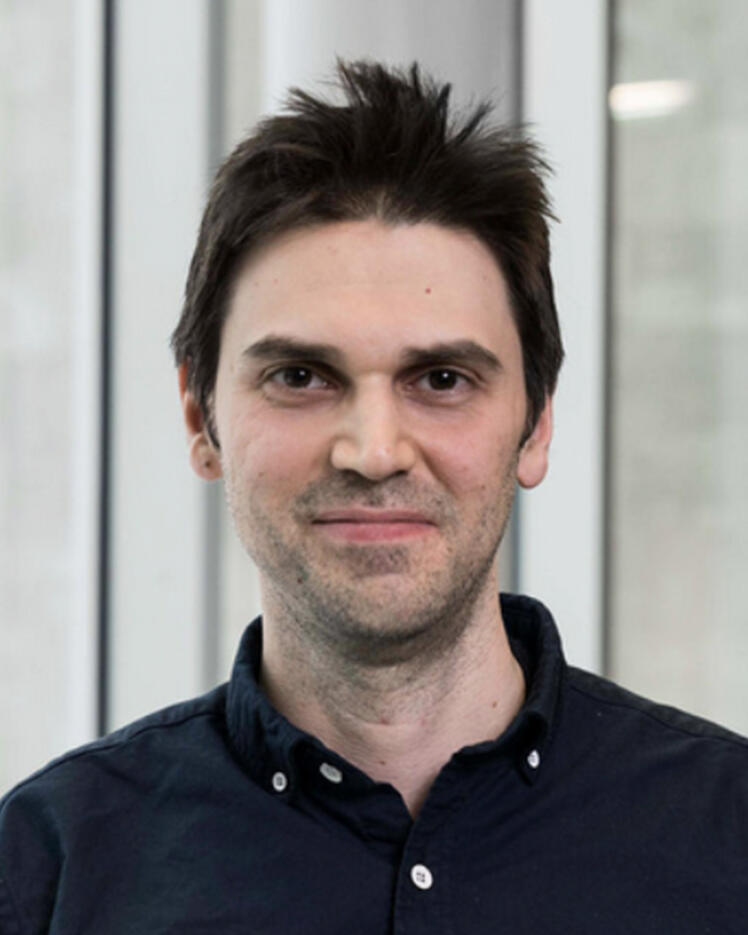
Cem (Yusuf) Subakan
Biographie
Cem Subakan est professeur adjoint à l'Université Laval, au sein du Département d'informatique et de génie logiciel. Il est également professeur adjoint affilié au Département d'informatique et de génie logiciel de l'Université Concordia, ainsi que membre académique associé à Mila – Institut québécois d'intelligence artificielle. Il a obtenu un doctorat en informatique de l'Université de l'Illinois à Urbana-Champaign (UIUC) et a effectué un postdoctorat à Mila. Il agit en tant que relecteur pour plusieurs conférences, notamment NeurIPS, ICML, ICLR, ICASSP et MLSP, ainsi que pour des revues telles que IEEE Signal Processing Letters (SPL) et IEEE Transactions on Audio, Speech, and Language Processing (TASL). Ses recherches portent principalement sur l'apprentissage automatique appliqué à la parole et à l'audio. Plus précisément, il travaille sur l'apprentissage profond pour la séparation de sources et l'amélioration de la parole dans des conditions réalistes, l'interprétabilité des réseaux neuronaux, l'apprentissage continu et l'apprentissage multimodal. Il a reçu le Prix du meilleur article étudiant lors de la conférence IEEE Machine Learning for Signal Processing (MLSP) en 2017, ainsi que la bourse Sabura Muroga du Département d'informatique de l'UIUC. Il est également un contributeur clé au projet SpeechBrain, où il dirige la partie consacrée à la séparation de la parole.