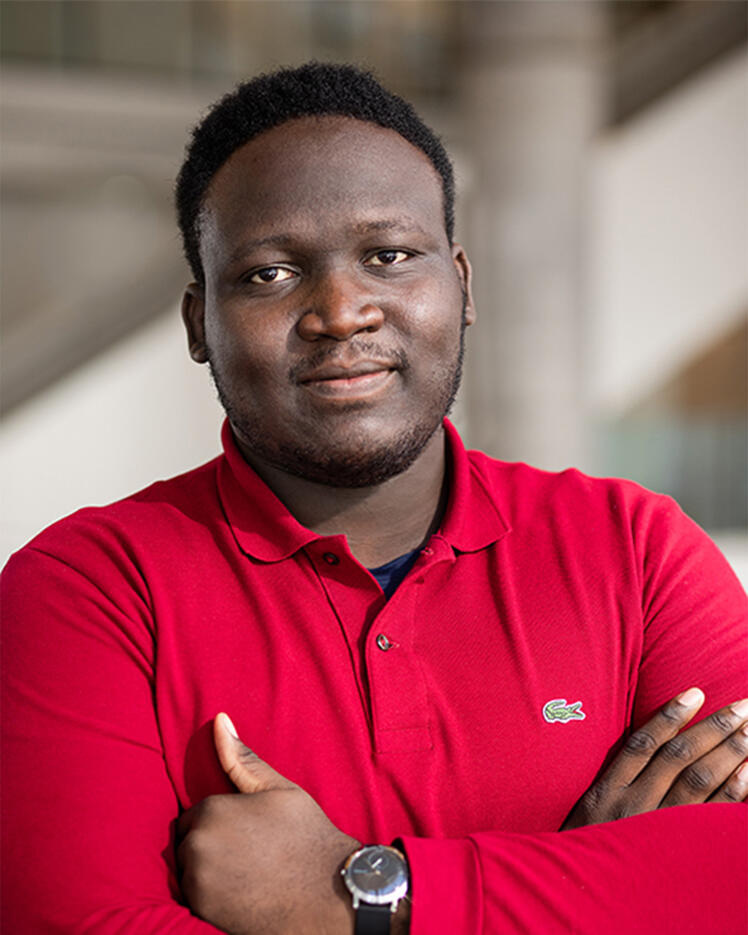
Ulrich Aivodji
Biographie
Ulrich Aivodji est professeur associé d'informatique au Département de génie logiciel et des technologies de l'information de l’École de technologie supérieure de Montréal (ÉTS).
Il dirige le Trustworthy Information Systems Lab (TISL). Ses domaines de recherche sont la sécurité informatique, la confidentialité des données, l'optimisation et l'apprentissage automatique. Ses travaux actuels portent sur plusieurs aspects de l'apprentissage automatique digne de confiance, tels que l'équité, l'apprentissage automatique préservant la vie privée et l'explicabilité.
Avant d'occuper son poste actuel, il était chercheur postdoctoral à l'Université du Québec à Montréal (UQAM), où il travaillait avec Sébastien Gambs sur l'éthique de l'apprentissage automatique et la protection de la vie privée. Il a obtenu un doctorat en informatique à l'Université Paul-Sabatier, sous la direction de Marie-José Huguet et Marc-Olivier Killijian. Pendant son doctorat, il a été affilié au Laboratoire de recherche spécialisé dans l’analyse et l’architecture des systèmes du Centre national de la recherche scientifique (LAAS-CNRS) en tant que membre des groupes de recherche Informatique fiable et tolérance aux fautes et Recherche opérationnelle, optimisation combinatoire et contraintes.