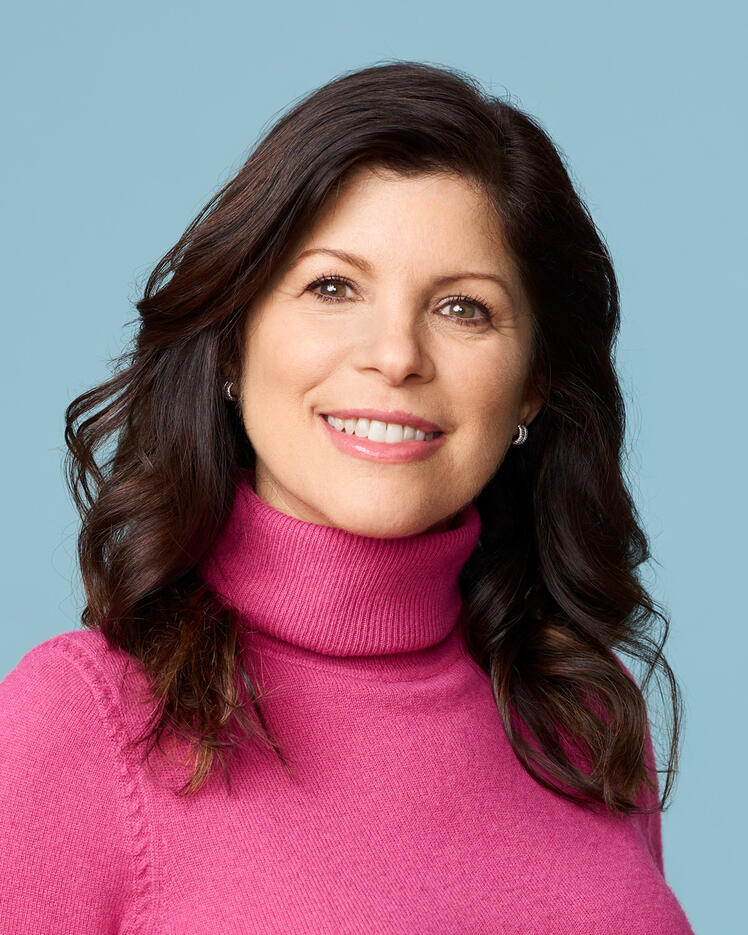
Tal Arbel
Biographie
Tal Arbel est professeure titulaire au Département de génie électrique et informatique de l’Université McGill, où elle dirige le groupe de vision probabiliste et le laboratoire d'imagerie médicale du Centre sur les machines intelligentes.
Elle est titulaire d'une chaire en IA Canada-CIFAR et membre associée de Mila – Institut québécois d’intelligence artificielle ainsi que du Centre de recherche sur le cancer Goodman. Les recherches de la professeure Arbel portent sur le développement de méthodes probabilistes d'apprentissage profond dans les domaines de la vision par ordinateur et de l’analyse d'imagerie médicale pour un large éventail d'applications dans le monde réel, avec un accent particulier sur les maladies neurologiques.
Elle a remporté le prix de la recherche Christophe Pierre 2019 de McGill Engineering. Elle fait régulièrement partie de l'équipe organisatrice de grandes conférences internationales sur la vision par ordinateur et l'analyse d'imagerie médicale (par exemple celles de la Medical Image Computing and Computer-Assisted Intervention Society/MICCAI et de Medical Imaging with Deep Learning/MIDL, l’International Conference on Computer Vision/ICCV ou encore la Conference on Computer Vision and Pattern Recognition/CVPR). Elle est rédactrice en chef et cofondatrice de la revue Machine Learning for Biomedical Imaging (MELBA).