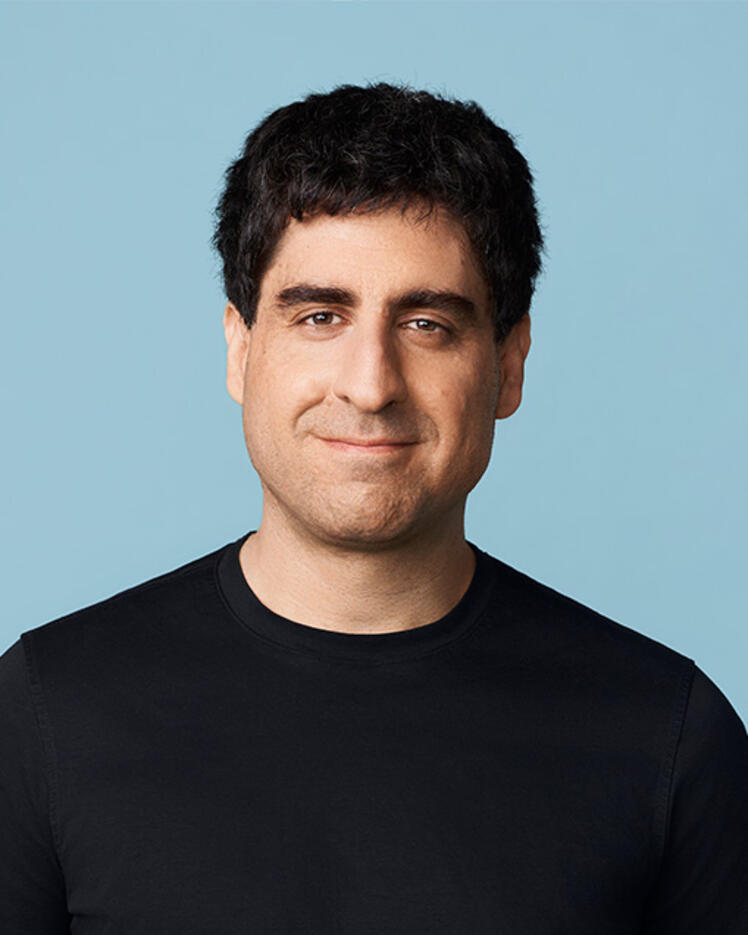
Siamak Ravanbakhsh
Biographie
Siamak Ravanbakhsh est professeur adjoint à l’École d’informatique de l’Université McGill depuis août 2019. Avant de se joindre à McGill et à Mila – Institut québécois d’intelligence artificielle, il a occupé un poste similaire à l’Université de la Colombie-Britannique. De 2015 à 2017, il a été stagiaire postdoctoral au Département d’apprentissage automatique et à l’Institut de robotique de l’Université Carnegie Mellon, et il a obtenu un doctorat de l’Université de l’Alberta. Il s’intéresse aux problèmes de l’apprentissage de la représentation et de l’inférence dans l’IA.
Ses recherches actuelles portent sur le rôle de la symétrie et de l’invariance dans l’apprentissage profond des représentations.