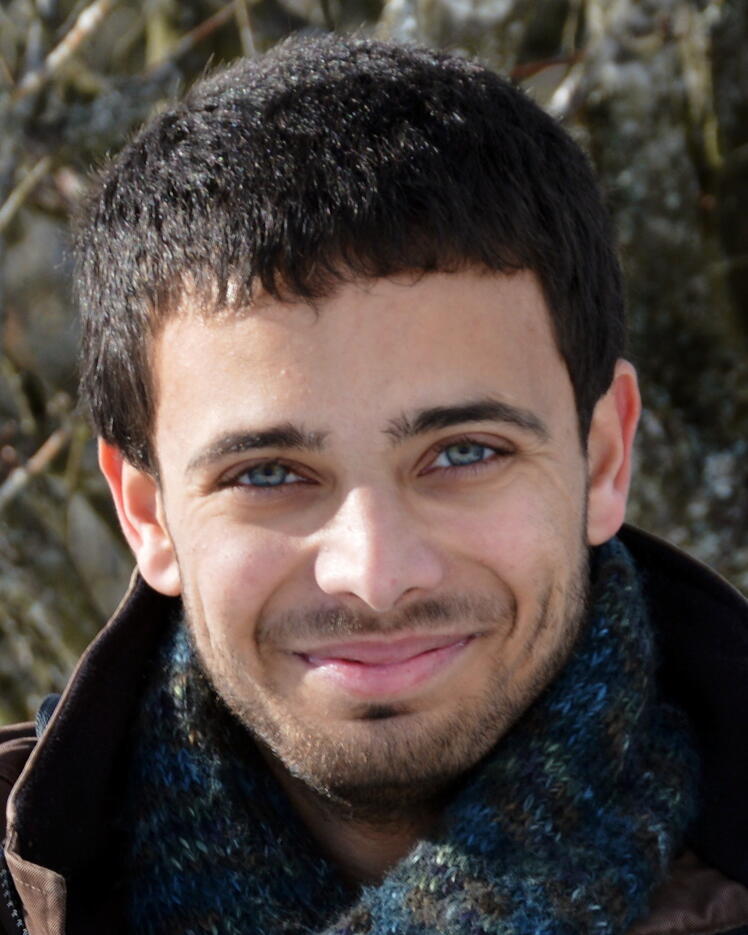
Quentin Cappart
Biographie
Quentin Cappart est professeur agrégé au Département de génie informatique et logiciel de Polytechnique Montréal et un membre affilié de Mila. Il a obtenu successivement un baccalauréat en ingénierie (2012), une M. Sc. en génie informatique (2014), une M. Sc. en gestion (2018) et un doctorat (2017) à l'Université catholique de Louvain (Belgique). Après son doctorat, il s’est joint à Polytechnique Montréal et au Centre interuniversitaire de recherche sur les réseaux d’entreprise, la logistique et le transport (CIRRELT) en tant que chercheur postdoctoral de 2018 à 2020. Pendant ces deux années, il a également été stagiaire en recherche chez ElementAI. Il dirige le groupe de recherche CORAIL, qu'il a cofondé avec le professeur Louis-Martin Rousseau. Ses recherches portent principalement sur l'intégration de l'apprentissage automatique à des procédures de recherche pour résoudre des problèmes combinatoires.