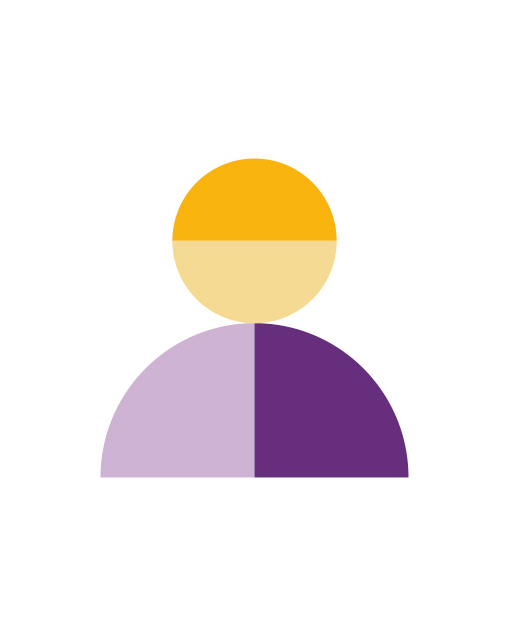
Gregory Dudek
Biographie
Gregory Dudek est professeur au Centre sur les machines intelligentes (CIM) de l’École d’informatique de l’Université McGill et directeur de recherche du Laboratoire de robotique mobile de l’université. Il est également chef de laboratoire et vice-président de la recherche du Centre d’intelligence artificielle de Samsung à Montréal.
Il a écrit, seul ou en collaboration, plus de 300 articles de recherche sur des sujets tels que la description et la reconnaissance d’objets visuels, la localisation de radiofréquences (RF), la navigation et la cartographie robotiques, la conception de systèmes distribués, les télécommunications 5G et la perception biologique. Il a notamment publié le livre Computational Principles of Mobile Robotics, en collaboration avec Michael Jenkin, aux éditions Cambridge University Press. Il a présidé ou a contribué à de nombreuses conférences et activités professionnelles nationales et internationales dans les domaines de la robotique, de la détection par machine et de la vision par ordinateur. Ses recherches portent sur la perception pour la robotique mobile, la navigation et l’estimation de la position, la modélisation de l’environnement et des formes, la vision informatique et le filtrage collaboratif.