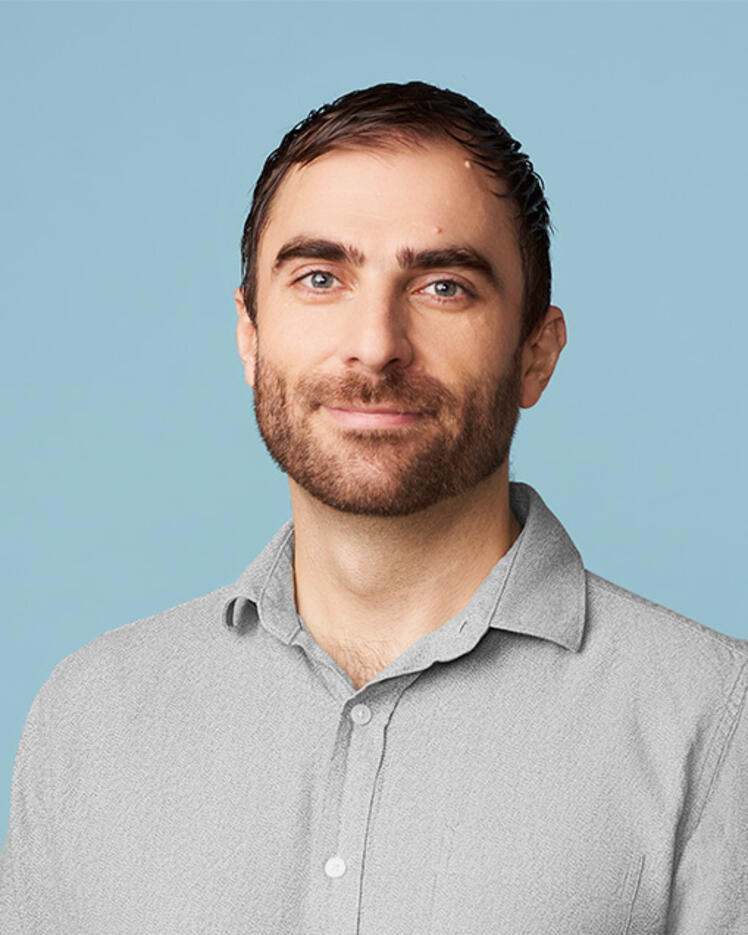
Glen Berseth
Biographie
Glen Berseth est professeur agrégé au Département d'informatique et de recherche opérationnelle (DIRO) de l'Université de Montréal, membre académique principal de Mila – Institut québécois d'intelligence artificielle, détenteur d’une chaire en IA Canada-CIFAR et codirecteur du Laboratoire de robotique et d’IA intégrative de Montréal (REAL). Il a été chercheur postdoctoral à Berkeley Artificial Intelligence Research (BAIR), où il a travaillé avec Sergey Levine. Ses recherches portent sur la résolution de problèmes de prise de décision séquentielle (planification) pour les systèmes d'apprentissage autonomes du monde réel (robots). Elles ont couvert les domaines de la collaboration humain-robot, du renforcement, ainsi que de l'apprentissage continu, multiagent et hiérarchique et du méta-apprentissage. Glen Berseth a fait paraître des articles dans les meilleures publications des domaines de la robotique, de l'apprentissage automatique et de l'animation informatique. Il donne également un cours sur l'apprentissage des robots à l'Université de Montréal et à Mila, couvrant les recherches les plus récentes sur les techniques d'apprentissage automatique pour la création de robots généralistes.