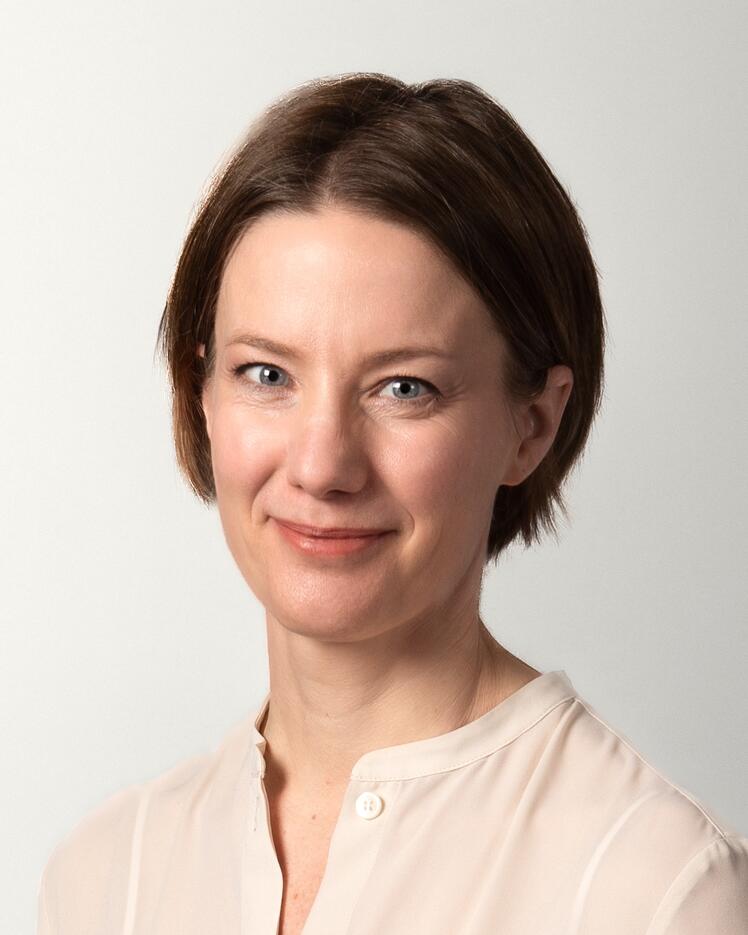
Emma Frejinger
Biographie
Emma Frejinger est professeure agrégée au Département d'informatique et de recherche opérationnelle (DIRO) de l'Université de Montréal, où elle est aussi titulaire d'une chaire de recherche du Canada et d'une chaire industrielle financée par la Compagnie des chemins de fer nationaux du Canada. Ses recherches sont axées sur les applications et se concentrent sur des combinaisons novatrices de méthodologies issues de l'apprentissage automatique et de la recherche opérationnelle pour résoudre des problèmes de prise de décision à grande échelle. Emma Frejinger possède une vaste expérience de travail avec l'industrie, en particulier dans le secteur des transports, où elle a dirigé des projets de recherche collaborative. Depuis 2018, elle est également conseillère scientifique pour IVADO Labs, où elle contribue au développement de solutions d'IA pour l'industrie de la chaîne d'approvisionnement. Avant de se joindre à l'Université de Montréal, en 2013, elle était membre du corps professoral de l'Institut royal de technologie KTH, en Suède. Elle est titulaire d'un doctorat en mathématiques de l'École polytechnique fédérale de Lausanne (EPFL), en Suisse.