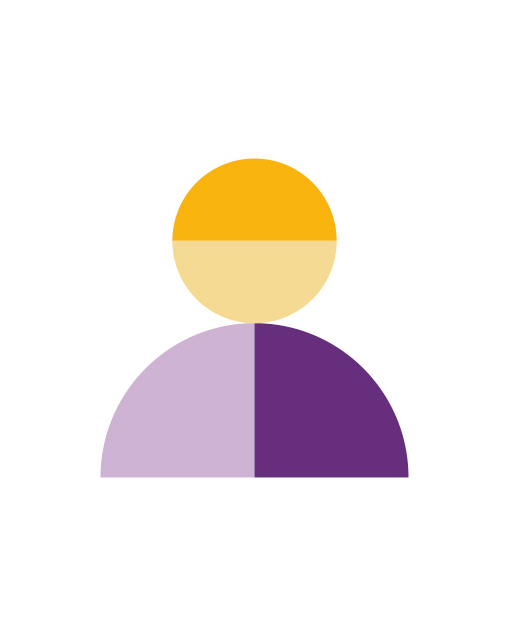
David Scott Krueger
Biographie
David Krueger est professeur adjoint en IA robuste, raisonnable et responsable au département d'informatique et de recherche opérationnelle (DIRO) et un membre académique principal à Mila - Institut québécois d'intelligence artificielle, au Center for Human-Compatible AI (CHAI) de l'université de Berkeley et au Center for the Study of Existential Risk (CSER). Ses travaux portent sur la réduction du risque d'extinction de l'humanité par l'intelligence artificielle (x-risque IA) par le biais de la recherche technique ainsi que de l'éducation, de la sensibilisation, de la gouvernance et de la défense des droits humains.
Ses recherches couvrent de nombreux domaines de l'apprentissage profond, de l'alignement de l'IA, de la sécurité de l'IA et de l'éthique de l'IA, notamment les modes de défaillance de l'alignement, la manipulation algorithmique, l'interprétabilité, la robustesse et la compréhension de la manière dont les systèmes d'IA apprennent et se généralisent. Il a été présenté dans les médias, notamment dans l'émission Good Morning Britain d'ITV, Inside Story d'Al Jazeera, France 24, New Scientist et l'Associated Press.
David a terminé ses études supérieures à l'Université de Montréal et à Mila - Institut québécois d'intelligence artificielle, où il a travaillé avec Yoshua Bengio, Roland Memisevic et Aaron Courville.