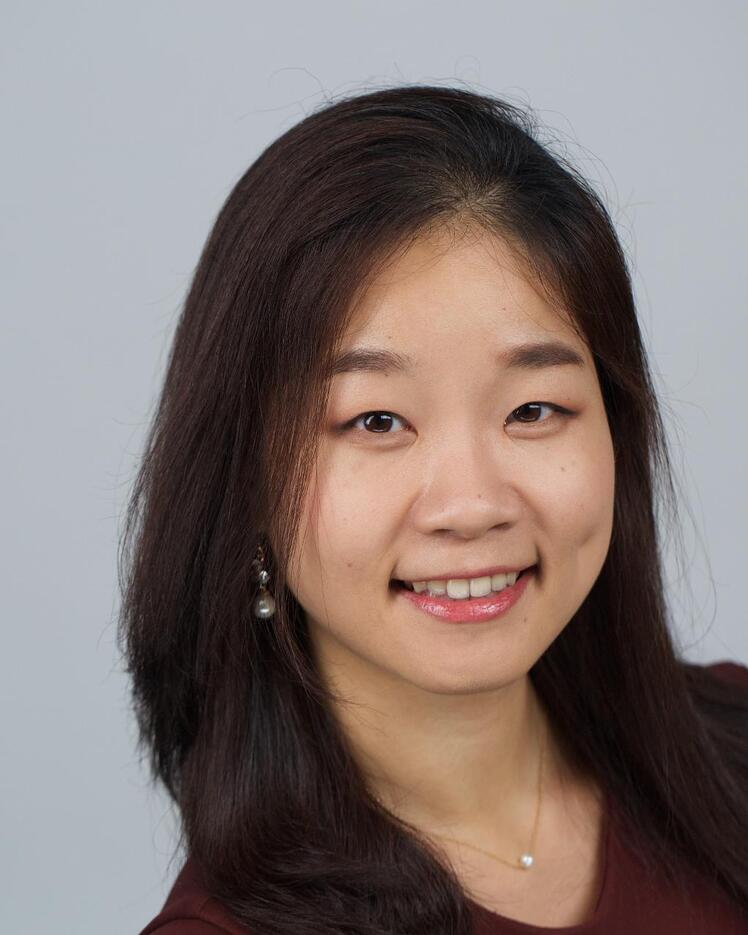
AJung Moon
Biographie
AJung Moon est une roboticienne expérimentale. Elle étudie comment les robots et les systèmes d'intelligence artificielle influencent la façon dont les gens se déplacent, se comportent et prennent des décisions, afin de déterminer comment nous pouvons concevoir et déployer de tels systèmes intelligents autonomes de manière plus responsable.
À l'Université McGill, elle est directrice du laboratoire McGill Responsible Autonomy & Intelligent System Ethics (RAISE). Le laboratoire RAISE est un groupe interdisciplinaire qui étudie les implications sociales et éthiques des robots et des systèmes d'intelligence artificielle, et explore ce que cela signifie pour les ingénieurs de concevoir et de déployer ces systèmes de manière responsable pour un avenir technologique meilleur.