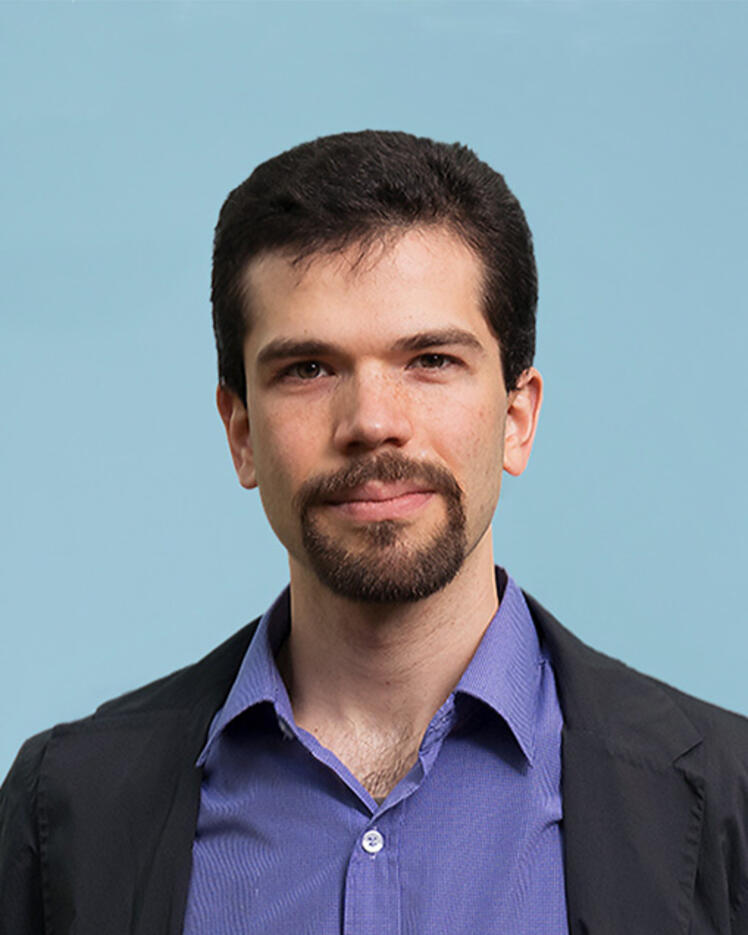
David Rolnick
Biography
David Rolnick is an assistant professor at McGill University’s School of Computer Science, a core academic member of Mila – Quebec Artificial Intelligence Institute and holds a Canada CIFAR AI Chair. Rolnick’s work focuses on applications of machine learning to help address climate change. He is the co-founder and chair of Climate Change AI, and scientific co-director of Sustainability in the Digital Age. After completing his PhD in applied mathematics at the Massachusetts Institute of Technology (MIT), he was a NSF Mathematical Sciences Postdoctoral Research Fellow, an NSF Graduate Research Fellow and a Fulbright Scholar. He was named to MIT Technology Review’s “35 Innovators Under 35” in 2021.