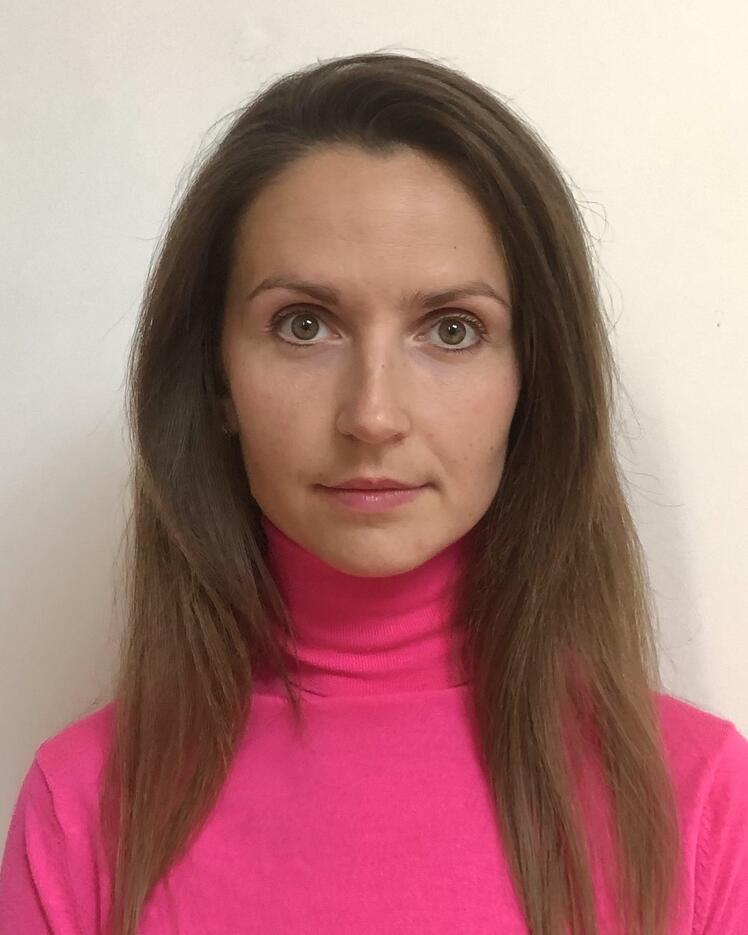
Gintare Karolina Dziugaite
Biography
Gintare Karolina Dziugaite is a senior research scientist at Google DeepMind in Toronto, and an adjunct professor at the McGill University School of Computer Science. Prior to joining Google, she led the Trustworthy AI program at Element AI (ServiceNow). Her research combines theoretical and empirical approaches to understanding deep learning.
Dziugaite is well known for her work on network and data sparsity, developing algorithms and uncovering effects on generalization and other metrics. She pioneered the study of linear mode connectivity, first connecting it to the existence of lottery tickets, then to loss landscapes and the mechanism of iterative magnitude pruning. Another major focus of her research is understanding generalization in deep learning and, more generally, the development of information-theoretic methods for studying generalization. Her most recent work looks at removing the influence of data on the model (unlearning).
Dziugaite obtained her PhD in machine learning from the University of Cambridge under the supervision of Zoubin Ghahramani. Prior to that, she studied mathematics at the University of Warwick and read Part III in Mathematics at the University of Cambridge, receiving a Master of Advanced Study (MASt) in mathematics. She has participated in a number of long-term programs at the Institute for Advanced Study in Princeton, NJ, and at the Simons Institute for the Theory of Computing at the University of Berkeley.